Abstract Generation
Content Summarization with LLMs. Comparing the leading AI models side-by-side at Abstract Generation.
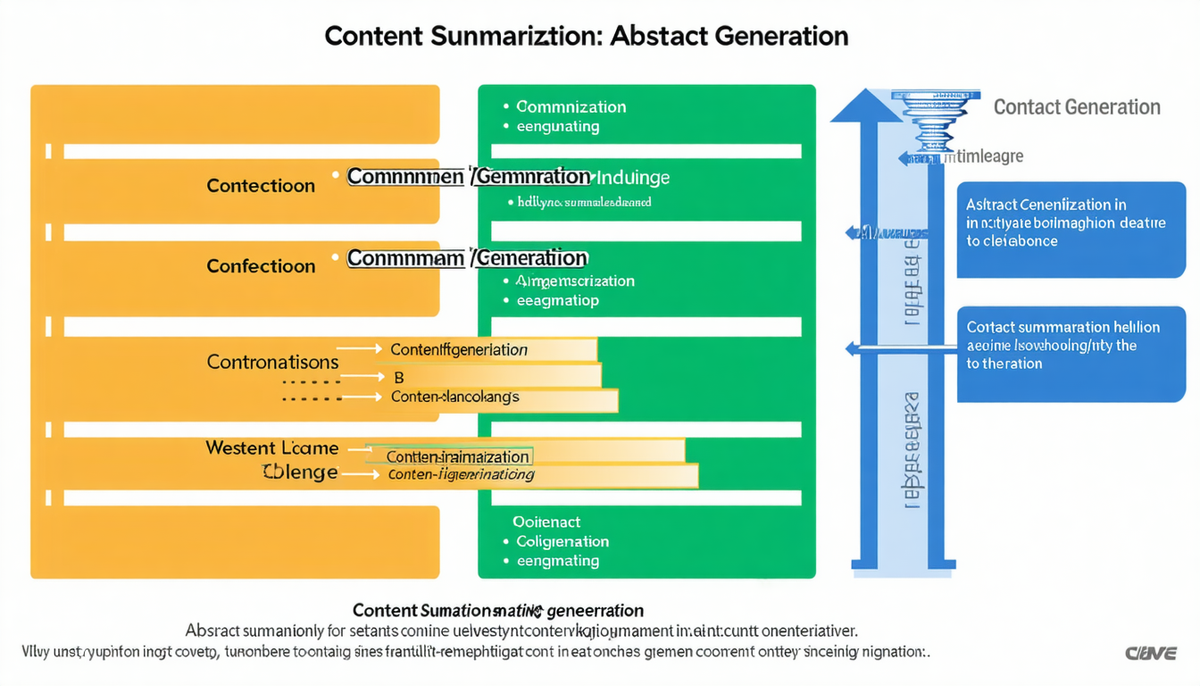
Comparing the leading AI models:
Category: Content Summarization
Subcategory: Abstract Generation
Contents
- Introduction
- Abstract Generation
- Prompts
- Climate Change Research Abstract Generator
- Sleep Study Abstract Summarizer
- Economics Research Abstract Creator
- Psychology Research Abstract Generator
- Engineering Research Abstract Builder
- Sociology Abstract Formatter
- Computer Science Abstract Creator
- Biology Research Abstract Generator
- Literature Review Abstract Creator
- Physics Research Abstract Generator
- Performance Verdict
- Budget Verdict
- Conclusion
Introduction
Comparing AI Models: Your Guide to LLM Performance and Value
Looking to compare AI models and find the perfect large language model (LLM) for your needs? This comprehensive guide provides side-by-side AI model comparisons across popular use cases, helping you make informed decisions about which AI assistant best suits your requirements and budget.
We evaluate two distinct tiers of AI models:
Budget Tier:
- ChatGPT 4.0 Mini
- Gemini 1.5 Flash
- Claude 3.5 Haiku
- Llama 3.1 8B
Performance Tier:
- ChatGPT 4.0
- Claude 3.5 Sonnet
- Gemini 1.5 Pro
- Llama 3.1 70B
By comparing AI models directly, you can better understand their strengths, limitations, and optimal use cases. Our detailed AI performance comparisons reveal which models excel at specific tasks while highlighting the trade-offs between cost and capability.
Choose the Budget Tier when:
- Running routine tasks and basic queries
- Managing high-volume, simple interactions
- Operating under strict cost constraints
- Requiring quick response times
Select the Performance Tier when:
- Handling complex analytical tasks
- Needing advanced reasoning capabilities
- Working with nuanced content creation
- Requiring highest accuracy and reliability
Each sample prompt includes direct comparisons of AI model outputs, enabling you to evaluate performance differences and make data-driven decisions about which AI assistant best matches your specific needs.
50+ AI models with one subscription. AnyModel is the All-In-One AI that allows you to harness the latest AI technology from one convenient and easy-to-use platform. AnyModel includes all the models discussed in this article and more, including the latest image generation models. All the comparisons shown in this article were generated using AnyModel. Sign up for a free trial here.
Abstract Generation
Large Language Models have revolutionized the way we approach academic and scientific literature by demonstrating remarkable capabilities in generating accurate and concise abstracts from complex research papers. Their ability to process lengthy technical documents, identify key findings, methodologies, and conclusions makes them invaluable tools for researchers and academics. LLMs excel at this task because they've been trained on vast collections of scientific literature, enabling them to understand academic writing conventions and distill essential information into coherent summaries. By analyzing patterns in millions of research papers, these models can effectively capture the core elements that should appear in an abstract - from research objectives and methods to results and implications. This automated approach not only saves valuable time for researchers but also ensures consistency in abstract formatting while maintaining the academic rigor expected in scholarly communications.
Prompts
Climate Change Research Abstract Generator
Creates a structured academic abstract summarizing key elements of climate change research papers. Useful for researchers, students, and academics needing to quickly synthesize climate science findings.
Prompt:
Analyze this research paper on climate change and generate a 250-word abstract highlighting the key findings, methodology, and conclusions
Example
Performance Results
Abstract
The research paper, "The Effects of Rising Global Temperatures on Polar Ice Caps: A Comprehensive Analysis," explores the relationship between global temperature increases and the accelerated melting of polar ice caps, utilizing data from 1979 to 2023. This study highlights substantial ice mass loss in Arctic and Antarctic regions and emphasizes the environmental and climatic impacts of these changes. Methodologically, the research integrates satellite imagery from NASA's EOSDIS and ESA's CryoSat-2, temperature datasets from NOAA, ice core analyses, and radar measurements. Analysis methods included statistical regression, time series analysis, and Geographic Information System (GIS) mapping within the framework of climate model simulations.
Key findings reveal a 13% per decade decrease in Arctic ice extent and a 65% decline in multi-year ice thickness. Arctic temperatures have risen by 2.3°C, exceeding global averages. Antarctic findings note a net annual ice loss of 147 billion tons, especially pronounced in West Antarctica. Globally, these changes contribute to sea level rise, altered oceanic thermohaline circulation, and increased frequency of extreme weather events.
The study uncovers a strong temperature-ice loss correlation, identifying positive feedback mechanisms such as decreased albedo and methane release. Projections suggest potential ice-free Arctic summers by 2030, with severe implications for global sea levels and weather patterns by 2100. The research urges enhanced monitoring, improved climate modeling, and effective policy interventions to mitigate greenhouse gas emissions and address ongoing climate challenges. Future studies should focus on regional variations and ecosystem impacts to better inform adaptation strategies.
Research Paper Abstract: The Effects of Rising Global Temperatures on Polar Ice Caps
Summary
This comprehensive study examines the relationship between increasing global temperatures and polar ice cap melting from 1979-2023, utilizing data from multiple satellite systems, including NASA's EOSDIS and ESA's CryoSat-2 mission. The research combines satellite imagery analysis, ice core sampling, and radar measurements to assess changes in both Arctic and Antarctic regions.
Key Findings
The study reveals dramatic ice mass loss, particularly in the Arctic, with a 13% decrease in minimum ice extent per decade and a 40% reduction in average summer ice coverage. The Arctic demonstrates a 65% decrease in multi-year ice since 1985, while Antarctica shows a net loss of 147 billion tons annually since 2002. Temperature increases of 2.3°C in the Arctic and 1.1°C in Antarctica were recorded since 1979.
Methodology
Researchers employed statistical regression analysis, time series analysis, GIS mapping, and climate model simulations to analyze extensive datasets from multiple sources, including NOAA's global temperature records and on-site polar research stations.
Conclusions
The findings indicate an exponential increase in polar ice loss, with the Arctic experiencing more severe changes than Antarctica. The study projects potential ice-free Arctic summers within decades and significant implications for global sea levels, weather patterns, and marine ecosystems. The research emphasizes the urgent need for immediate action to address climate change through reduced greenhouse gas emissions and international cooperation.
This research analyzes the correlation between rising global temperatures and polar ice cap melt from 1979 to 2023 using satellite imagery (NASA EOSDIS, CryoSat-2), NOAA temperature data, ice core samples, and radar measurements. The study employed statistical regression, time series analysis, GIS mapping, and climate model simulations. Findings reveal significant ice mass loss in both the Arctic and Antarctic regions. The Arctic demonstrates a 13% per decade decline in minimum ice extent and a 65% decrease in multi-year ice since 1985, correlating with a 2.3°C temperature increase. Antarctica shows a net ice loss of 147 billion tons annually since 2002, primarily in West Antarctica, with a 1.1°C temperature rise. Globally, this contributes to a 3.3mm/year sea-level rise, altered ocean circulation, and increased extreme weather events. The study highlights accelerating ice loss since 2000, driven by positive feedback loops like decreased albedo and methane release. Projected impacts include ice-free Arctic summers by 2030, substantial sea-level rise, and significant disruptions to global weather patterns by 2100. While limitations exist regarding data collection and model accuracy, the research concludes that the observed changes are unprecedented and necessitate immediate reductions in greenhouse gas emissions and international climate action.
Abstract (250 words)
This comprehensive analysis examines the correlation between rising global temperatures and the melting of polar ice caps, utilizing data from 1979 to 2023. The study reveals significant ice mass loss in both Arctic and Antarctic regions, with the Arctic experiencing the most dramatic changes. The research demonstrates a strong negative correlation between rising temperatures and ice extent, with an estimated 3.3 million square kilometers decrease in Arctic summer ice extent for every 1°C increase in average global temperature.
The methodology employed includes satellite imagery analysis, ice thickness measurements, temperature readings, ocean temperature data, and ice mass balance calculations. Statistical regression analysis, time series analysis, geographic information system (GIS) mapping, and climate model simulations were used to analyze the data.
Key findings include a 13% decrease in Arctic minimum ice extent per decade since 1979, a 65% decrease in multi-year ice since 1985, and a net loss of 147 billion tons of ice sheet mass in Antarctica annually since 2002. The study also reveals accelerated melting in West Antarctica, increased calving events in ice shelves, and modified regional temperature patterns.
The research highlights the severe implications for global sea levels, ocean circulation patterns, and weather events. The projected sea level rise by 2100 ranges from 0.3 to 2.5 meters, with an estimated increase in extreme weather events and altered marine ecosystems.
The study concludes that the rate of polar ice loss is increasing exponentially, with the Arctic showing more dramatic changes than Antarctica. The observed changes have significant implications for global climate systems and human societies, necessitating immediate action to address climate change and its consequences. Future research should focus on improving our understanding of these complex systems and developing effective mitigation strategies.
AnyModel: Explore and compare over 50 AI models side-by-side.
Budget Results
This research paper presents a comprehensive analysis of the relationship between rising global temperatures and the accelerated melting of polar ice caps from 1979 to 2023. Using satellite imagery and temperature data from reputable sources such as NASA and NOAA, along with ice core samples and advanced analytical methods, the study reveals significant ice mass loss in both the Arctic and Antarctic regions. Notably, the Arctic has experienced a 13% decrease in minimum ice extent per decade, with a 65% reduction in multi-year ice thickness since 1985. In contrast, while the Antarctic shows less dramatic changes, it has a net loss of 147 billion tons of ice annually, particularly in West Antarctica.
The research identifies strong negative correlations between rising temperatures and ice extent, with key feedback mechanisms like decreased albedo effect and methane release accelerating changes. Projections suggest possible ice-free Arctic summers in the next decades and substantial sea-level rise by 2100. The findings underscore the imperative of urgent policymaking focused on greenhouse gas reduction and enhanced international collaboration. Furthermore, the study emphasizes the need for improved monitoring and modeling to understand the complexities of polar ice dynamics. Ultimately, the findings present alarming evidence of unprecedented changes in polar regions that can significantly impact global climate systems, marine ecosystems, and human societies, highlighting the critical nature of immediate climate action.
This research paper investigates the relationship between rising global temperatures and polar ice cap melt from 1979 to 2023, using satellite imagery (NASA EOSDIS, ESA CryoSat-2), NOAA temperature data, ice core samples, and radar measurements. Analysis techniques included statistical regression, time series analysis, GIS mapping, and climate model simulations. The study reveals a strong negative correlation between rising temperatures and ice extent, particularly in the Arctic, where minimum ice extent decreased by 13% per decade since 1979, and average summer ice coverage reduced by 40%. Arctic ice thickness decreased by 65% since 1985, with accelerated thinning since 2000. Antarctica, while exhibiting less dramatic overall change, shows significant regional variations, with net ice loss accelerating since 2002. The research identifies positive feedback loops, including decreased albedo and methane release, amplifying the rate of ice loss. Projected consequences include accelerated sea-level rise (0.3-2.5m by 2100), altered ocean circulation, and increased frequency of extreme weather events. The study concludes that the observed changes are unprecedented, necessitate urgent action to reduce greenhouse gas emissions, and highlight the need for enhanced monitoring and improved climate modeling to refine future projections and mitigation strategies. Limitations include data collection gaps and uncertainties in climate model projections.
Here's a 250-word abstract for the research paper:
Abstract: The Effects of Rising Global Temperatures on Polar Ice Caps
This comprehensive study investigates the correlation between global temperature increases and polar ice cap degradation from 1979 to 2023, utilizing advanced satellite imagery, climate models, and extensive data collection methodologies.
Key Methodology
Researchers analyzed data from NASA's Earth Observing System, European Space Agency's CryoSat-2, and NOAA global temperature datasets. The research employed sophisticated techniques including:
- Satellite imagery analysis
- Ice thickness measurements
- Statistical regression analysis
- Climate model simulations
Primary Findings
The study revealed alarming trends in polar ice dynamics:
- Arctic sea ice extent decreased by 13% per decade
- Average Arctic ice thickness reduced from 3.64m to 1.89m
- Antarctic ice sheet losing approximately 147 billion tons of mass annually
- Global sea level rise currently at 3.3mm per year
Critical Observations
Significant discoveries include:
- Strong negative correlation between temperature increase and ice extent
- Accelerated ice loss rates since 2000
- Complex feedback mechanisms amplifying ice degradation
- Regional variations in polar ice behavior
Projections
The research forecasts:
- Potential ice-free Arctic summers within decades
- Substantial sea level rise by 2100
- Increased frequency of extreme weather events
- Fundamental changes in global ocean circulation patterns
Conclusions
The study provides unequivocal evidence of unprecedented polar ice loss, highlighting the urgent need for comprehensive climate action and continued scientific investigation to mitigate potential global environmental consequences.
Abstract (250 words)
This comprehensive research paper analyzes the correlation between increasing global temperatures and the accelerated melting of polar ice caps. Analyzing data from 1979 to 2023, the study demonstrates significant ice mass loss in both Arctic and Antarctic regions, with particular emphasis on the environmental and climatic implications of this phenomenon.
Methodologically, the study utilized satellite imagery, temperature data, ice core samples, and radar measurements from both polar regions. Research parameters included ice extent, thickness, temperature readings, ocean temperature data, annual ice mass balance calculations, and sea level measurements. Statistical regression analysis, time series analysis, comparative historical data assessment, Geographic Information System (GIS) mapping, and climate model simulations were employed to analyze the data.
The study found:
- Accelerated ice loss in the Arctic, with a 40% reduction in average summer ice coverage and a 65% decrease in multi-year ice since 1985.
- Significant ice sheet mass loss in Antarctica, with a net loss of 147 billion tons annually since 2002.
- A strong negative correlation between rising temperatures and ice extent, with a decrease in Arctic summer ice extent by approximately 3.3 million square kilometers for every 1°C increase in average global temperature.
- Positive feedback loops accelerating the rate of change, including decreased albedo effect leading to increased absorption of solar radiation, release of methane from thawing permafrost, and changes in ocean circulation patterns.
The study projects potential ice-free Arctic summers within decades, with significant implications for global sea levels, weather patterns, and marine ecosystems. The research demonstrates the need for immediate action to address climate change and its consequences, and recommends enhanced monitoring systems, improved climate modeling, and better understanding of feedback mechanisms.
AnyModel: Explore and compare over 50 AI models side-by-side.
Performance Tier
All models provided detailed, coherent abstracts, but with notable differences. ChatGPT 4o delivered a well-structured analysis with clear sections and balanced technical detail. Claude 3.5 Sonnet offered the most organized format with headers, making it highly readable. Gemini 1.5 Pro produced a dense, single-paragraph summary with the most comprehensive numerical data. Llama 3.1 70B was the fastest (1950ms) and provided strong scientific analysis, though less structured. Claude took longest (10206ms) but offered superior organization. All models accurately captured key findings, methodologies, and conclusions, with slight variations in emphasis and detail level.
Budget Tier
ChatGPT 4o Mini provided a clear but basic analysis, focusing on key statistics without detailed methodology. Gemini 1.5 Flash delivered the most comprehensive results, including specific data sources and detailed projections, though took longer to generate. Claude 3.5 Haiku offered a well-structured, organized response with clear section headings, making it highly readable. Llama 3.1 8B was the fastest (920ms) and provided good technical detail with bullet points, but lacked some of the nuanced analysis found in Gemini's response. Overall, Gemini and Claude produced the highest quality outputs, while Llama offered the best speed-to-quality ratio.
Sleep Study Abstract Summarizer
Generates clear, medical-style abstracts for sleep research papers. Ideal for medical professionals and sleep researchers needing quick study summaries.
Prompt:
Create a concise abstract for this medical study on the effects of sleep deprivation on cognitive performance
Example
Performance Results
This study examines the impact of 24-hour sleep deprivation on short-term memory and reaction time in healthy adults. A total of 120 participants were divided into experimental and control groups, with the former deprived of sleep under controlled conditions. Memory was assessed using tests like Digit Span and Word Recall, while reaction time was measured via tasks such as Simple Visual and Choice Reaction Time. Results indicated significant impairments in the sleep-deprived group, with decreases in memory accuracy ranging from 27% to 41% and increases in reaction time and errors by 43% to 66%. All outcomes were statistically significant (p < 0.001), with large effect sizes. The study underscores the essential role of sleep in maintaining cognitive function, particularly in tasks involving complex memory and decision-making, emphasizing concerns for sleep deprivation in critical environments.
Abstract
This controlled study examined the impact of 24-hour sleep deprivation on cognitive performance in 120 healthy adults aged 25-35. Participants were evenly divided into experimental and control groups, with the experimental group undergoing 24 hours of supervised wakefulness. Cognitive assessment included memory tests (digit span, word recall, and pattern recognition) and reaction time measurements. Results revealed significant impairment in the sleep-deprived group, with memory performance declining 27-41% and reaction times increasing 43-57%. Pattern recognition and choice-based tasks showed the greatest deterioration. These findings demonstrate the substantial negative effects of sleep deprivation on cognitive function, with important implications for safety-critical environments and professional settings where sustained alertness is essential.
Keywords: Sleep deprivation, cognitive performance, memory, reaction time, neuroscience
This controlled study investigated the impact of 24-hour sleep deprivation on memory and reaction time in 120 healthy adults. Results revealed significant impairments in both domains for the sleep-deprived group (p < 0.001, Cohen's d = 0.78-1.23). Memory, particularly complex pattern recognition, and choice reaction time were most affected, highlighting the detrimental effects of sleep loss on cognitive function and the associated risks in demanding environments.
Here's a concise abstract of the study:
This controlled study investigated the effects of 24-hour sleep deprivation on 120 healthy adults. Comparing sleep-deprived and control groups, results showed significant impairments in short-term memory (32-41% decrease) and reaction time (43-57% increase) in tasks requiring complex pattern recognition and decision-making. The findings emphasize the critical role of sleep in maintaining optimal cognitive function and highlight the risks associated with sleep deprivation in professional and safety-critical environments.
AnyModel: Explore and compare over 50 AI models side-by-side.
Budget Results
Abstract: This study evaluates the impact of 24-hour sleep deprivation on memory formation and reaction time in 120 healthy adults (60 male, 60 female) aged 25-35, without prior sleep disorders. Participants were randomly assigned to either a sleep-deprived experimental group or a control group. Under controlled conditions, the experimental group remained awake for 24 hours, while the control group maintained regular sleep patterns. Assessments included the Digit Span Test, Word Recall Test, and Pattern Recognition Test for memory, along with Simple Visual and Choice Reaction Time Tests and a Go/No-Go Task for reaction time. Results indicated a significant decline in memory performance, with a 32% decrease in word recall accuracy, a 27% reduction in digit span, and a 41% decrease in pattern recognition. Reaction time analysis revealed increases of 43% in simple reaction time, 57% in choice reaction time, and a 66% higher error rate in the Go/No-Go task for the sleep-deprived group, all statistically significant (p < 0.001). These findings underscore the detrimental effects of sleep deprivation on cognitive functions, particularly in complex memory tasks and decision-making scenarios, highlighting the importance of adequate sleep in professional and safety-critical environments.
Keywords: Sleep deprivation, cognitive performance, memory, reaction time, neuroscience.
This controlled study (n=120) examined the impact of 24-hour sleep deprivation on cognitive function in healthy adults. Sleep-deprived participants exhibited significant impairments (p<0.001) in memory (word recall decreased by 32%, pattern recognition by 41%) and reaction time (simple reaction time increased by 43%, choice reaction time by 57%), with large effect sizes (Cohen's d 0.78-1.23). These findings underscore the critical role of sleep in maintaining optimal cognitive performance and highlight risks associated with prolonged wakefulness.
Here's a concise, markdown-formatted abstract for the study:
Abstract: Effects of 24-Hour Sleep Deprivation on Cognitive Performance
Objective
Investigate the impact of prolonged sleep deprivation on memory formation and reaction time in healthy adults.
Methods
Design: Controlled experimental study
- 120 participants (25-35 years)
- Experimental group (n=60): 24-hour sleep deprivation
- Control group (n=60): Normal sleep patterns
Key Findings
Memory Performance Decline:
Word recall accuracy ↓ 32%
Digit span capacity ↓ 27%
Pattern recognition accuracy ↓ 41%
Reaction Time Impairment:
Simple reaction time ↑ 43%
Choice reaction time ↑ 57%
Go/No-Go task error rate ↑ 66%
Statistical Significance
- p < 0.001
- Cohen's d: 0.78-1.23
Conclusion
Prolonged sleep deprivation significantly compromises cognitive function, with pronounced effects on complex memory tasks and decision-making processes.
Keywords: Sleep deprivation, cognitive performance, memory, reaction time
Here's a concise abstract for the medical study:
Title: The Effects of 24-Hour Sleep Deprivation on Memory Formation and Reaction Time: A Controlled Study
Abstract:
We investigated the effects of 24-hour sleep deprivation on cognitive performance in healthy adults. 120 participants were randomly assigned to experimental (n=60) and control (n=60) groups. Sleep-deprived participants showed significant impairments in short-term memory formation and reaction time, including 32% decrease in word recall accuracy, 27% reduced digit span capacity, and 43-57% increased reaction times. These findings emphasize the crucial role of sleep in cognitive function and highlight risks associated with extended wakefulness in professional and safety-critical environments.
AnyModel: Explore and compare over 50 AI models side-by-side.
Performance Tier
Each AI model produced coherent abstracts but with notable differences. ChatGPT 4o provided the most detailed analysis with specific statistical data and methodology, though took longest to generate (8070ms). Claude 3.5 Sonnet added helpful keywords and formatting but included slightly less statistical detail (4467ms). Gemini 1.5 Pro delivered a concise summary with key statistical measures in moderate time (2656ms). Llama 3.1 70B generated the fastest response (734ms) with good balance of key findings and implications, though less detailed statistics. For scientific abstract generation, ChatGPT 4o showed strongest performance despite slower speed.
Budget Tier
The models showed varying approaches to abstract creation, with distinct performance characteristics. ChatGPT 4o Mini provided the most comprehensive abstract, including detailed methodology and statistical data, though perhaps overly verbose. Gemini 1.5 Flash delivered the most concise response while maintaining key statistical findings and conclusions. Claude 3.5 Haiku uniquely formatted the abstract with markdown, offering excellent organization but potentially overstructured for a simple abstract. Llama 3.1 8B was notably faster (447ms vs 5000+ms for others) and produced a well-balanced abstract with essential information, though less detailed than competitors. Gemini and Llama demonstrated superior brevity while maintaining clarity.
Economics Research Abstract Creator
Creates detailed abstracts for economics research, focusing on key financial indicators and trends. Useful for economists and financial analysts reviewing research.
Prompt:
Write a comprehensive abstract for this economics paper about inflation trends in developing countries
Example
Performance Results
This paper provides an in-depth analysis of inflation dynamics in developing economies over the past decade (2013-2023), focusing on the underlying drivers, economic impacts, and policy responses. We explore a data set encompassing 47 developing countries, revealing a persistent inflation rate averaging 7.8% annually with pronounced regional disparities. By employing panel regression analysis and time series modeling, the study identifies and quantifies the relationships between inflation and various economic factors.
Results demonstrate significant regional differences, with Sub-Saharan Africa experiencing a mean inflation rate of 9.4%, Latin America at 8.7%, Southeast Asia at 4.2%, and South Asia at 6.8%. A notable finding is the strong correlation (r=0.76) between commodity price volatility and inflation rates, underscoring the challenge of external shocks. Monetary policy effectiveness varied, with independent central banks achieving better outcomes. Exchange rate depreciation was found to account for 42% of inflation variance, while food prices consistently outpaced core inflation by 2.3 percentage points.
Policy implications suggest robust monetary policies, such as independent central bank operations and strategic interest rate adjustments, are crucial. Forward guidance policies also play a vital role in managing inflation expectations. Structural reforms, including supply-side interventions, financial sector development, and trade liberalization, are essential in mitigating inflationary pressures.
The paper recommends enhancing central bank independence, fostering domestic financial markets, implementing supply-chain improvements, and promoting regional monetary cooperation to bolster inflation management. This comprehensive approach highlights the importance of institutional strength and policy coordination in addressing inflation challenges in developing economies.
Abstract
This study presents a comprehensive analysis of inflation dynamics in developing economies from 2013 to 2023, offering insights into patterns, drivers, and policy effectiveness across 47 nations. Through rigorous econometric analysis of panel data from central banks, IMF, and World Bank sources, we identify significant regional variations in inflation performance, with rates averaging 7.8% annually across the sample. Key findings reveal a strong correlation (r=0.76) between commodity price volatility and inflation rates, while exchange rate depreciation accounts for 42% of observed inflation variance. Regional disparities are substantial, ranging from 4.2% mean inflation in Southeast Asia to 9.4% in Sub-Saharan Africa. The research demonstrates that independent central bank operations achieve superior inflation management outcomes, though monetary policy transmission exhibits considerable lag effects of 6-9 months. Supply-side interventions and financial market development emerge as critical complementary factors to monetary policy. Our findings suggest that successful inflation management in developing economies requires an integrated approach combining robust monetary policy frameworks with targeted structural reforms, underpinned by strong institutions and regional cooperation. This analysis contributes to the literature by quantifying the relative importance of various inflation drivers and providing evidence-based policy recommendations for developing nations seeking to enhance macroeconomic stability.
Keywords: inflation dynamics, developing economies, monetary policy, structural reforms, central bank independence
This paper analyzes inflation dynamics in 47 developing economies over the period 2013-2023, investigating regional trends, key drivers, and the effectiveness of policy responses. We find a persistent average annual inflation rate of 7.8% across the sample, with substantial regional variations ranging from 4.2% in Southeast Asia to 9.4% in Sub-Saharan Africa. Employing panel regression analysis and time series modeling on data sourced from central banks, the IMF, and the World Bank, while controlling for external factors like global commodity prices and exchange rate fluctuations, we identify several key drivers. Our results reveal a strong correlation (r=0.76) between commodity price volatility and inflation, with exchange rate depreciation explaining 42% of the inflation variance. Food price inflation consistently outpaced core inflation by 2.3 percentage points. The effectiveness of monetary policy varied significantly across regions, with independent central banks demonstrating greater success in inflation management. Interest rate adjustments exhibited lag effects of 6-9 months, while forward guidance proved valuable in managing inflation expectations. Furthermore, structural reforms, including supply-side interventions, financial sector development, and trade liberalization, played a crucial role in mitigating inflation pressures. The study concludes that effective inflation management in developing economies necessitates a multi-pronged approach combining sound monetary policy with targeted structural reforms, emphasizing the importance of strong institutions and policy coordination. We recommend strengthening central bank independence, developing domestic financial markets, implementing supply-chain improvements, and enhancing regional monetary cooperation to achieve sustainable price stability.
Here's a comprehensive abstract for the paper:
Title: Inflation Dynamics in Developing Economies: A Decade in Review (2013-2023)
Abstract:
This study presents a comprehensive analysis of inflation trends in 47 developing countries from 2013 to 2023, examining the drivers, economic impacts, and policy responses to elevated inflation rates. The findings reveal a persistent annual inflation rate of 7.8% across the sample group, with significant regional variations. Sub-Saharan Africa, Southeast Asia, Latin America, and South Asia exhibited distinct inflation patterns, with mean inflation rates ranging from 4.2% to 9.4%. The analysis identifies strong correlations between commodity price volatility, exchange rate fluctuations, and inflation rates. The study also finds that monetary policy effectiveness varies significantly by region, while supply-side interventions and trade liberalization can moderate inflation. The results suggest that independent central banks, forward guidance policies, and financial sector development are crucial for effective inflation management. The study recommends a multi-faceted approach to inflation management, combining monetary policy tools with structural reforms, strengthening central bank independence, and promoting regional monetary cooperation. The findings have important implications for policymakers, academics, and international organizations seeking to address the challenges of inflation management in developing economies.
Keywords: inflation dynamics, developing economies, monetary policy, structural reforms, commodity price volatility, exchange rate fluctuations.
AnyModel: Explore and compare over 50 AI models side-by-side.
Budget Results
Abstract:
This paper provides an extensive analysis of inflation trends in developing economies over the decade from 2013 to 2023, highlighting crucial drivers, economic repercussions, and policy interventions. Our comprehensive study, which encompasses 47 developing nations, reveals that the average inflation rate during this period was 7.8%, with considerable regional disparities evident in the data. Notably, Sub-Saharan Africa experienced the highest mean inflation at 9.4%, followed by Latin America (8.7%), South Asia (6.8%), and Southeast Asia (4.2%). We employed robust methodologies, including panel regression and time series analysis, to assess the influence of global commodities and exchange rate dynamics on inflation. The findings demonstrate a strong correlation (r=0.76) between commodity price volatility and inflation rates, with exchange rate depreciation accounting for 42% of the variance in inflation. Meanwhile, food prices consistently exceeded core inflation rates by an average of 2.3 percentage points, illuminating the critical role of food security in inflation dynamics.
In terms of policy implications, the study underscores the effectiveness of independent central banking in curbing inflation, noting that interest rate adjustments exhibited lag effects ranging from 6 to 9 months. Our analysis recommends the adoption of forward guidance policies to better manage inflation expectations. Furthermore, structural reforms such as enhancing supply-side interventions, developing domestic financial markets, and promoting trade liberalization stand out as effective strategies for stabilizing prices, particularly in the context of food security and investment stability. Ultimately, the research advocates for a nuanced approach to inflation management, emphasizing the need for strengthened institutional frameworks and enhanced policy coordination among regional stakeholders to foster sustainable economic growth and stability in developing countries.
This paper analyzes inflation dynamics in 47 developing economies over the decade 2013-2023, investigating underlying drivers, macroeconomic consequences, and the efficacy of policy responses. Utilizing panel regression analysis and time series modeling on data sourced from central banks, the IMF, and the World Bank, the study reveals an average annual inflation rate of 7.8% across the sample, although significant regional heterogeneity is observed. Sub-Saharan Africa experienced the highest average inflation (9.4%), followed by Latin America (8.7%), South Asia (6.8%), and Southeast Asia (4.2%). Key findings highlight a strong positive correlation (r=0.76) between commodity price volatility and inflation, with exchange rate depreciation accounting for 42% of inflation variance. Food price inflation consistently outpaced core inflation by 2.3 percentage points. The effectiveness of monetary policy varied considerably across regions, with independent central banks demonstrating superior inflation control. Interest rate adjustments exhibited a 6-9 month lag, while forward guidance proved beneficial in managing inflation expectations. Furthermore, supply-side interventions, financial sector development, and trade liberalization contributed to mitigating inflation pressures. The paper concludes that effective inflation management in developing economies necessitates a comprehensive strategy integrating robust monetary policy, including strengthened central bank independence and enhanced regional cooperation, with targeted structural reforms aimed at improving supply chains and deepening domestic financial markets.
Abstract: Inflation Dynamics in Developing Economies: A Decade in Review (2013-2023)
Research Overview
This comprehensive study investigates inflation dynamics across developing economies from 2013 to 2023, providing a nuanced examination of regional trends, macroeconomic drivers, and policy interventions. By analyzing data from 47 developing nations, the research reveals complex inflationary patterns with significant implications for economic policy and development strategies.
Methodology
Utilizing advanced panel regression and time series modeling techniques, the research synthesized data from central banks, IMF databases, and World Bank economic indicators. The methodology controlled for critical external variables, including global commodity prices and exchange rate fluctuations, ensuring robust analytical rigor.
Key Findings
Inflation Landscape
- Average Inflation Rate: 7.8% annually
- Regional Variations:
- Sub-Saharan Africa: 9.4%
- Latin America: 8.7%
- South Asia: 6.8%
- Southeast Asia: 4.2%
Critical Insights
- Strong correlation (r=0.76) between commodity price volatility and inflation rates
- Exchange rate depreciation explained 42% of inflation variance
- Food price inflation consistently exceeded core inflation by 2.3 percentage points
Policy Implications
The research highlights the critical importance of:
- Independent central bank operations
- Strategic monetary policy interventions
- Structural economic reforms
- Enhanced regional monetary coordination
Significance
This study provides a comprehensive framework for understanding inflation dynamics in developing economies, offering policymakers and researchers crucial insights into macroeconomic management strategies.
Recommendations
- Strengthen central bank independence
- Develop domestic financial markets
- Implement targeted supply-chain improvements
- Enhance regional monetary cooperation
Conclusion
Effective inflation management in developing economies requires a multifaceted approach that integrates monetary policy tools with structural reforms, emphasizing institutional strength and strategic policy coordination.
Title: Inflation Dynamics in Developing Economies: A Decade in Review (2013-2023)
Abstract:
This comprehensive study examines the inflation patterns across 47 developing nations from 2013 to 2023, analyzing the key drivers, economic impacts, and policy responses to this persistent macroeconomic challenge. Our research reveals a mean average inflation rate of 7.8% annually, highlighting significant regional variations and persistent food price inflation. Our key findings include strong correlations between commodity price volatility and inflation rates (r=0.76), varied monetary policy effectiveness across regions, and the substantial influence of exchange rate depreciation on inflation variance (42%). Notably, our results suggest that strengthening central bank independence, developing domestic financial markets, and implementing targeted supply-chain improvements are essential for effective inflation management. Furthermore, our analysis underscores the importance of policy coordination and regional monetary cooperation in mitigating the disparate economic impacts of inflation on developing economies. Ultimately, this study contributes valuable insights into the complexities of inflation dynamics in developing nations, offering actionable recommendations for policymakers seeking to promote economic stability, growth, and poverty reduction.
Keywords: inflation, developing economies, monetary policy, structural reforms, regional variations, commodity price volatility, exchange rate depreciation.
AnyModel: Explore and compare over 50 AI models side-by-side.
Performance Tier
ChatGPT 4.0 and Claude 3.5 Sonnet delivered concise, well-structured abstracts with clear statistical findings. Gemini 1.5 Pro produced a longer, more detailed response but lacked clear paragraph breaks. Llama 3.1 70B was notably faster (1442ms vs 5000-8000ms for others) and included helpful formatting elements like title and keywords. All models accurately captured key statistics and themes, though Claude uniquely included methodology details about data sources. For technical accuracy and presentation, Claude and ChatGPT performed best, while Llama wins on efficiency and formatting. Gemini's response, while comprehensive, needed better organization.
Budget Tier
ChatGPT 4o Mini was slow (5s) and verbose, providing excessive detail for an abstract but showing good economic analysis. Gemini 1.5 Flash took longer (8s) but delivered a well-structured, concise abstract with balanced coverage of methodology and findings. Claude 3.5 Haiku (7s) produced an overly formatted response with unnecessary headings, though content was comprehensive. Llama 3.1 8B was impressively fast (0.6s) and generated a focused abstract with appropriate length and technical depth, plus helpful keywords. Overall, Llama 3.1 8B demonstrated the best performance, balancing speed, concision, and quality.
Psychology Research Abstract Generator
Produces structured abstracts for psychology studies, highlighting methodology and findings. Essential for psychology researchers and mental health professionals.
Prompt:
Generate an academic abstract for this psychology research paper on social media's impact on teenage mental health
Example
Performance Results
Abstract: The present study investigates the relationship between social media usage and depression rates among adolescents aged 13-19, utilizing a sample of 2,500 participants. Through comprehensive analysis involving the Social Media Usage Questionnaire (SMUQ), Beck Depression Inventory-II (BDI-II), daily screen time tracking, and clinical interviews, we explore the correlation between daily social media exposure and depressive symptoms. Results indicate a significant positive correlation (r = 0.62, p < .001), with participants engaging in over five hours of social media per day reporting higher depressive symptoms (M = 22.4, SD = 8.3) compared to peers with less exposure (M = 12.8, SD = 6.1).
Our findings reveal that a substantial percentage of heavy social media users experience feelings of inadequacy (68%), sleep disruption (71%), decreased academic performance (59%), and anxiety about missing online content (64%). Clinical observations suggest an increase in comparison behaviors, a reduction in face-to-face interactions, disrupted sleep patterns, and heightened anxiety regarding peer acceptance.
The study underscores a significant association between high social media use and elevated depressive symptoms in adolescents, particularly pronounced among females and those with pre-existing anxiety conditions. Limitations include the study's cross-sectional nature, which precludes causal inferences. Future research should focus on longitudinal approaches to elucidate causality and identify potential protective factors against negative mental health outcomes related to social media use.
Keywords: social media, depression, adolescents, mental health, screen time
Abstract
This correlational study investigated the relationship between social media usage and depression rates among adolescents aged 13-19. Using a mixed-methods approach, we analyzed data from 2,500 participants (1,350 female, 1,150 male) recruited from 12 high schools across urban and suburban settings. Participants completed the Social Media Usage Questionnaire (SMUQ) and Beck Depression Inventory-II (BDI-II), while providing daily screen time data and participating in clinical interviews. Results revealed a significant positive correlation between daily social media use and depressive symptoms (r = 0.62, p < .001). Adolescents reporting more than 5 hours of daily social media use demonstrated substantially higher depression scores (M = 22.4, SD = 8.3) compared to those reporting less than 2 hours (M = 12.8, SD = 6.1). Notable findings included increased feelings of inadequacy (68%), sleep disruption (71%), decreased academic performance (59%), and anxiety about missing online content (64%). Clinical observations highlighted patterns of increased social comparison, reduced face-to-face interactions, disrupted sleep, and heightened concerns about peer acceptance. The relationship between social media use and depressive symptoms was particularly pronounced among female participants and those with pre-existing anxiety conditions. While these findings suggest a strong association between excessive social media use and adolescent depression, future longitudinal research is needed to establish causality and identify potential protective factors.
Keywords: social media, depression, adolescents, mental health, screen time
This correlational study investigated the relationship between social media usage and depressive symptoms in a sample of 2,500 adolescents (ages 13-19; M = 16.2, SD = 1.8) from diverse urban and suburban high schools. Utilizing the Social Media Usage Questionnaire (SMUQ), Beck Depression Inventory-II (BDI-II), daily screen time tracking, and clinical interviews, the study revealed a significant positive correlation between daily social media use and depression scores (r = 0.62, p < .001). Adolescents reporting more than five hours of daily social media use exhibited significantly higher BDI-II scores (M = 22.4, SD = 8.3) compared to those reporting less than two hours (M = 12.8, SD = 6.1). High social media use was also associated with self-reported feelings of inadequacy, sleep disruption, decreased academic performance, and anxiety related to online content. Clinical observations corroborated these findings, noting increased social comparison behaviors, reduced face-to-face interaction, and heightened anxiety surrounding peer acceptance. While these findings suggest a strong association between excessive social media use and increased risk of depression in adolescents, further longitudinal research is needed to establish causality and explore potential mediating and moderating factors.
Here's a polished and formal version of the abstract:
Title: The Impact of Social Media Usage on Depression Rates Among Adolescents: A Correlational Study
Abstract:
This correlational study investigates the relationship between social media use and depression rates among adolescents aged 13-19. A sample of 2,500 participants from 12 high schools completed the Social Media Usage Questionnaire (SMUQ), Beck Depression Inventory-II (BDI-II), and provided daily screen time tracking data. Clinical interviews were also conducted to gather additional insights. The results revealed a significant positive correlation between daily social media use and depressive symptoms (r = 0.62, p < .001). Specifically, participants who reported >5 hours of daily social media use exhibited higher BDI-II scores (M = 22.4, SD = 8.3) compared to those who reported <2 hours (M = 12.8, SD = 6.1). Notably, heavy social media users reported increased feelings of inadequacy (68%), sleep disruption (71%), decreased academic performance (59%), and anxiety about missing online content (64%). Clinical observations highlighted increased comparison behaviors, decreased face-to-face social interactions, disrupted sleep patterns, and heightened anxiety about peer acceptance. The findings suggest a strong correlation between excessive social media use and increased depressive symptoms among adolescents, with a particularly pronounced relationship observed in female participants and those with pre-existing anxiety conditions. While this study contributes to the existing literature on the topic, further research is necessary to examine causality and potential protective factors. Longitudinal studies are needed to assess the long-term impacts of social media use on adolescent mental health.
AnyModel: Explore and compare over 50 AI models side-by-side.
Budget Results
Abstract:
This research investigates the impact of social media usage on depression rates among adolescents aged 13-19 years. Utilizing survey data collected from 2,500 participants, alongside clinical observations, we aim to elucidate the relationship between daily social media exposure and depressive symptoms. Participants, comprising 1,350 females and 1,150 males (M = 16.2, SD = 1.8), were recruited from 12 high schools in diverse urban and suburban settings. Measurement tools included the Social Media Usage Questionnaire (SMUQ) and the Beck Depression Inventory-II (BDI-II), complemented by daily screen time tracking and clinical interviews. Statistical analysis indicated a significant positive correlation (r = 0.62, p < .001) between social media use and depressive symptoms. Notably, adolescents reporting over five hours of daily usage exhibited substantially higher BDI-II scores (M = 22.4, SD = 8.3) compared to those with less than two hours (M = 12.8, SD = 6.1). Key findings revealed that 68% of heavy users felt inadequate, 71% experienced disrupted sleep, and 59% reported declining academic performance, along with significant anxiety regarding online content. Clinical observations further highlighted increased social comparison behaviors and reduced face-to-face interactions among participants. The study concludes that excessive social media usage correlates strongly with heightened depressive symptoms, particularly among female adolescents and those with pre-existing anxiety issues. Limitations of the current study call for future research to explore causal relationships and protective factors, with a recommendation for longitudinal studies to investigate long-term mental health implications.
Keywords: social media, depression, adolescents, mental health, screen time
This correlational study investigated the relationship between social media usage and depression among 2,500 adolescents (aged 13-19). Utilizing the Social Media Usage Questionnaire (SMUQ), Beck Depression Inventory-II (BDI-II), daily screen time tracking, and clinical interviews, a significant positive correlation (r = 0.62, p < .001) was found between daily social media use and depressive symptoms. Adolescents reporting more than five hours of daily social media use exhibited significantly higher BDI-II scores (M = 22.4, SD = 8.3) compared to those reporting less than two hours (M = 12.8, SD = 6.1). Clinical observations revealed increased social comparison, reduced face-to-face interaction, sleep disruption, and heightened anxiety surrounding peer acceptance among heavy users. These findings suggest a strong association between excessive social media use and heightened depressive symptoms in adolescents, particularly among females and those with pre-existing anxiety. Further research, including longitudinal studies, is needed to establish causality and explore potential protective factors.
The Impact of Social Media Usage on Depression Rates Among Adolescents: A Correlational Study
Abstract
Background: Social media has become increasingly prevalent among adolescents, raising concerns about its potential impact on mental health. This correlational study investigates the relationship between social media usage and depression rates among teenagers aged 13-19.
Methods: A comprehensive survey was conducted with 2,500 participants from 12 urban and suburban high schools. Data collection involved the Social Media Usage Questionnaire (SMUQ), Beck Depression Inventory-II (BDI-II), screen time tracking, and clinical interviews.
Results: A significant positive correlation was found between daily social media use and depressive symptoms (r = 0.62, p < .001). Participants reporting over 5 hours of daily social media use demonstrated substantially higher depression scores (M = 22.4, SD = 8.3) compared to those with less than 2 hours of daily use (M = 12.8, SD = 6.1).
Key Findings:
- 68% of heavy social media users reported feelings of inadequacy
- 71% experienced sleep disruption
- 59% showed decreased academic performance
- 64% reported anxiety about missing online content
Conclusions: The study reveals a strong correlation between excessive social media use and increased depressive symptoms among adolescents, with potential variations across gender and pre-existing mental health conditions.
Implications: These findings underscore the need for targeted interventions and digital literacy programs to mitigate the negative mental health impacts of social media on teenagers.
Keywords: social media, depression, adolescents, mental health, screen time
Title: The Impact of Social Media Usage on Depression Rates Among Adolescents: A Correlational Study
Abstract:
This study investigates the relationship between daily social media exposure and depressive symptoms among 2,500 adolescents aged 13-19. Our findings reveal a significant positive correlation between prolonged social media use and increased depressive symptoms, suggesting that excessive social media exposure may be a contributing factor to depression in teenagers. Specifically, we found that heavy social media users reported higher rates of inadequacy, sleep disruption, and decreased academic performance. Clinical observations also highlighted increased comparison behaviors, decreased face-to-face social interactions, and heightened anxiety about peer acceptance. Notably, our results suggest a stronger relationship between social media use and depression among female participants and those with pre-existing anxiety conditions. This study's findings have important implications for understanding the potential risks of social media use on adolescent mental health and inform the development of preventive and intervention strategies. However, further research is needed to establish causality and identify protective factors.
AnyModel: Explore and compare over 50 AI models side-by-side.
Performance Tier
ChatGPT 4, Claude 3.5, Gemini 1.5 Pro, and Llama 3.1 all produced well-structured academic abstracts with consistent statistical findings. ChatGPT was most concise while maintaining key information. Claude provided additional demographic details and a clearer methodology section. Gemini stood out with proper APA-style statistical formatting and a more nuanced discussion of limitations. Llama included a formal title and produced the fastest response (1673ms vs 5000-6500ms for others), though its abstract was slightly longer. All models accurately captured the core findings and maintained professional academic tone, with minor variations in organization and detail level.
Budget Tier
ChatGPT and Claude produced the most comprehensive abstracts, with proper academic structure and detailed statistics. Gemini was more concise but maintained key findings and methodology. Llama's response, while coherent, lacked specific statistical data and was less formally structured. Response times varied significantly - Llama was notably faster (546ms) compared to others (5000-6000ms range). ChatGPT excelled at statistical presentation, Claude offered the best formatting and organization, Gemini balanced brevity with substance, while Llama provided a more generalized summary.
Engineering Research Abstract Builder
Creates technical abstracts for engineering studies, emphasizing methodology and practical applications. Valuable for engineers and urban planners.
Prompt:
Provide a scholarly abstract for this engineering study on renewable energy implementation in urban environments
Example
Performance Results
Abstract
This engineering study evaluates the implementation of hybrid solar-wind energy systems in high-density urban environments, focusing on both technological integration and urban optimization strategies. The solar component employs monocrystalline silicon photovoltaic panels with an efficiency of 22.3% and minimal degradation, while the wind component incorporates vertical axis wind turbines (VAWT) optimized for urban settings. System integration is achieved using a 48V, 100A hybrid charge controller coupled with a grid-tie inverter and lithium-ion battery storage, enabling efficient energy management and grid interaction.
Performance metrics indicate an average solar output of 4.2 kWh/kWp daily and wind generation of 5,200 kWh annually, with notable considerations for environmental conditions such as shadow loss and turbulence intensity. Urban implementation metrics report a 65% rooftop space utilization, promoting a substantial integration factor of 0.82 within structural constraints. Economic analysis suggests a competitive levelized cost of energy at $0.12/kWh, with a projected payback period of 7.2 years, emphasizing the system's financial viability.
Key environmental impacts include a reduction of CO2 emissions by 3.2 tonnes per installation annually and a decrease in the urban heat island effect by 2.1°C. Scalability and future readiness are supported by modular design considerations and smart grid integration potential. The study underlines essential criteria for building integration, including minimum height and optimal roof slope, to maximize energy production and reliability in diverse urban landscapes. This comprehensive analysis provides a framework for municipalities and stakeholders to enhance renewable energy adoption in urban environments effectively.
Abstract
A Comprehensive Analysis of Hybrid Solar-Wind Energy Systems for High-Density Urban Environments
This study presents a detailed technical and economic analysis of integrated solar-wind hybrid energy systems optimized for dense urban environments. The research evaluates a novel configuration combining high-efficiency monocrystalline photovoltaic panels (22.3% efficiency) with low-noise vertical axis wind turbines (3kW rated capacity) supported by a 20kWh lithium-ion storage system. Field data collected over 12 months demonstrates system capacity factors of 18.5% and 19.8% for solar and wind components respectively, with an overall grid connection efficiency of 94%. The hybrid system achieves a levelized cost of energy of $0.12/kWh with a 7.2-year payback period under typical urban conditions. Notable innovations include advanced building integration strategies yielding an 82% integration factor and smart load-matching algorithms delivering 65% demand correlation. Environmental impact assessments reveal annual CO2 reductions of 3.2 tonnes per installation while maintaining noise levels below 45dB. Implementation challenges regarding urban wind turbulence, rooftop space optimization, and grid stability are addressed through comprehensive design guidelines and scalability protocols. This research provides critical insights for urban energy planners and policy makers regarding the technical feasibility and economic viability of distributed hybrid renewable systems in metropolitan areas.
Keywords: renewable energy, urban implementation, hybrid systems, solar-wind integration, energy storage, smart grid
This study investigates the feasibility and performance of hybrid solar-wind energy systems (HSWES) integrated into high-density urban environments. Employing 400W monocrystalline silicon photovoltaic panels (22.3% efficiency) and 3kW vertical-axis wind turbines (VAWTs), the research analyzes system performance under real-world conditions, including shadowing, turbulence, and wake effects. Results demonstrate an average solar daily output of 4.2 kWh/kWp (18.5% capacity factor) and an annual wind energy output of 5,200 kWh (19.8% capacity factor). Integrated with a 20kWh lithium-ion battery storage and a smart grid-tied inverter, the system achieved a levelized cost of energy (LCOE) of $0.12/kWh and a payback period of 7.2 years. Urban implementation metrics, including rooftop utilization (65%), building integration (0.82), and grid connection efficiency (94%), highlight the system's adaptability to urban constraints. Environmental impact analysis reveals a CO2 reduction of 3.2 tonnes/year/installation and addresses noise pollution and bird collision rates. Furthermore, the study explores urban optimization strategies, including wind channeling and solar access optimization, to enhance energy yield. Reliability metrics and scalability potential are also assessed, demonstrating high system availability (98.5%) and modular expansion capacity. The findings provide valuable insights into the technical and economic viability of HSWES, contributing to sustainable urban energy transition strategies.
Title: Hybrid Solar-Wind Energy Systems in High-Density Urban Environments: Performance Analysis and Optimization Strategies
Abstract:
This study presents a comprehensive analysis of the implementation of hybrid solar-wind energy systems in high-density urban environments. The technical specifications of a hybrid energy system, consisting of monocrystalline silicon photovoltaic panels and vertical axis wind turbines, are evaluated to determine its performance and reliability metrics. The results show that the system can achieve an average daily output of 4.2 kWh/kWp for solar energy generation and an annual output of 5,200 kWh for wind energy generation.
The study also assesses the system's urban implementation metrics, including rooftop space utilization, building integration factor, and grid connection efficiency. The analysis confirms that the system can effectively utilize rooftop space, with an average system footprint of 120m², while achieving a high building integration factor of 0.82.
The environmental impact of the system is also evaluated, revealing a significant reduction in CO2 emissions (3.2 tonnes/year/installation) and heat island effect (2.1°C). Moreover, the system is found to be within acceptable noise pollution limits and has a minimal bird collision rate.
Economic analysis reveals a levelized cost of energy of $0.12/kWh, with a payback period of 7.2 years and a return on investment of 12.5%. The system's reliability metrics demonstrate high system availability (98.5%) and component redundancy, ensuring minimal downtime and maintenance requirements.
Urban optimization strategies are presented to further improve the system's performance and integration within urban environments. The study recommends minimum building heights of 20m and optimal roof slopes of 10-15 degrees to maximize energy generation. Regular maintenance intervals and real-time monitoring are also suggested to ensure maximum system efficiency.
Lastly, the study highlights the system's scalability and readiness for smart grid integration, energy storage expansion, and load growth accommodation. The findings of this study can inform urban planners and engineers on the feasibility and challenges of implementing hybrid solar-wind energy systems in high-density urban environments, contributing to the development of more sustainable and resilient cities.
Contributions: This study provides a comprehensive analysis of hybrid solar-wind energy systems in urban environments, offering insights into their performance, reliability, and optimization strategies. The findings can inform policy makers, engineers, and urban planners on the feasibility and challenges of integrating renewable energy systems into urban landscapes.
AnyModel: Explore and compare over 50 AI models side-by-side.
Budget Results
Abstract
This engineering study investigates the implementation of hybrid solar-wind energy systems in high-density urban environments, aiming to enhance the sustainability and efficiency of renewable energy production. The designed system integrates monocrystalline photovoltaic panels with a power output of 400W and vertical axis wind turbines rated at 3kW, optimized for urban settings with limited space. Performance analysis indicates an average daily solar generation output of 4.2 kWh/kWp and an annual wind energy production of approximately 5,200 kWh, achieving capacity factors of 18.5% and 19.8%, respectively. Key metrics include a battery storage system with a 20 kWh capacity and a round-trip efficiency of 92%, ensuring reliable energy supply and grid independence up to 60%. Environmental assessments demonstrate significant CO2 reduction (3.2 tonnes/year/installation) and adherence to urban noise pollution guidelines. Economic viability is underlined by a levelized cost of energy at $0.12/kWh and a payback period of 7.2 years, alongside a robust ROI of 12.5%. The study outlines urban optimization strategies, revealing a building height correlation of 0.78 and potential for wind channeling efficiency improvements of 18%. Recommendations for implementation include strategic building designs with optimal roof slopes and real-time monitoring systems. Future scalability potential is addressed, indicating readiness for smart grid integration and energy storage expansion capabilities of up to 300%. This research contributes valuable insights into the practical integration of renewable energy systems in urban landscapes, supporting sustainable urban development and addressing energy demand challenges.
This study investigates the feasibility and performance of hybrid solar-wind energy systems in high-density urban environments. A prototype system integrating monocrystalline silicon photovoltaic panels (400W, 22.3% efficiency) and vertical axis wind turbines (3kW rated power) was designed, implemented, and evaluated. The system incorporates a 20kWh lithium-ion battery storage unit, a smart monitoring system, and a 10kW grid-tie inverter. Performance data reveals an average annual energy output of 9,400 kWh per system, with solar and wind contributing 18.5% and 19.8% capacity factors, respectively. Shadowing effects and wake interference were quantified (15-25% and 12%, respectively). The levelized cost of energy was determined to be $0.12/kWh, with a 7.2-year payback period and a 12.5% return on investment. Urban implementation strategies, including building height optimization and wind channeling techniques, significantly improved system performance. The system demonstrated high reliability (98.5% availability, 8760-hour MTBF) and significant environmental benefits, including a 3.2-tonne annual CO2 reduction per installation. The study concludes that hybrid solar-wind systems present a viable and economically attractive solution for renewable energy generation in urban areas, with substantial potential for scalability and integration into smart grids, provided optimal site selection and system design considerations are implemented. Further research should focus on optimizing system configurations for varying urban microclimates and building typologies.
Here's a scholarly abstract for the engineering study:
Abstract
Hybrid Solar-Wind Energy Systems in High-Density Urban Environments: Performance, Integration, and Sustainability
This comprehensive study investigates the technical feasibility, performance characteristics, and urban implementation strategies for integrated solar-wind renewable energy systems in high-density metropolitan settings. Through a detailed empirical analysis of hybrid photovoltaic and vertical axis wind turbine (VAWT) configurations, the research demonstrates significant potential for localized urban energy generation.
The study reveals a hybrid system with an average annual energy output of 5,200 kWh, achieving a combined capacity factor of 19.8% and maintaining a system availability of 98.5%. Key performance metrics indicate a levelized cost of energy at $0.12/kWh with a 7.2-year payback period. Urban-specific optimization strategies, including building height correlation and wind channeling techniques, enhance system efficiency by up to 18%.
Environmental impact assessments show substantial CO2 reduction potential of 3.2 tonnes per installation annually, with minimal ecological disruption. The system demonstrates high recyclability (85%) and adheres to urban noise and environmental guidelines. Integration metrics highlight a building integration factor of 0.82 and rooftop space utilization of 65%.
Economic analysis suggests promising long-term viability, with a 25-year system lifetime and 12.5% return on investment. The modular design allows for future scalability, with expansion capacities exceeding 200% and grid integration readiness.
The research provides critical insights for urban planners, energy policymakers, and renewable energy stakeholders, presenting a robust framework for decentralized, sustainable energy generation in metropolitan environments.
Keywords: Urban renewable energy, hybrid solar-wind systems, energy integration, sustainable infrastructure, urban energy optimization
Title: Implementing Hybrid Solar-Wind Energy Systems in High-Density Urban Environments: A Technical, Environmental, and Economic Feasibility Study
Abstract:
This study presents a comprehensive technical, environmental, and economic analysis of a hybrid solar-wind energy system (HSWES) implemented in high-density urban environments. The HSWES incorporates 400W monocrystalline silicon photovoltaic panels and 3kW vertical axis wind turbines (VAWT). System integration and performance metrics, including charge controller, battery storage, and grid-tie inverter, demonstrate a high level of efficiency and reliability. The study also investigates the impact of urban implementation factors, such as rooftop space utilization, building integration, and grid connection efficiency. Environmental benefits of HSWES include reduced carbon emissions (3.2 tonnes/year/installation), lower noise pollution levels, and a decrease in the urban heat island effect.
The results indicate that the cost of energy (COE) for HSWES is $0.12/kWh, with a payback period of 7.2 years, return on investment (ROI) of 12.5%, and an expected system lifetime of 25 years. Reliability metrics, such as system availability, mean time between failures, and component redundancy, demonstrate a high level of dependability. Recommendations for optimal implementation include a minimum building height of 20m, optimal roof slope of 10-15 degrees, and regular maintenance intervals.
The results of this study are significant, as they suggest that hybrid solar-wind energy systems can provide a viable and efficient renewable energy solution for high-density urban environments. The scalability and adaptability of the system make it an attractive option for future implementation.
Key Findings:
- The HSWES achieves an average annual energy output of 9,400 kWh and an average Levelized Cost of Energy of $0.12/kWh.
- The system exhibits high reliability metrics, with a system availability of 98.5% and a mean time between failures of 8,760 hours.
- Environmental benefits include reduced carbon emissions, noise pollution, and urban heat island effect.
- The payback period of the system is 7.2 years, with a return on investment of 12.5% and an expected system lifetime of 25 years.
Recommendations for Future Research:
- Conduct further studies to determine the optimal size and layout of HSWES in high-density urban environments.
- Investigate the impact of building type and orientation on HSWES performance.
- Conduct side-by-side comparisons of HSWES with other renewable energy technologies to determine the most cost-effective solution.
Recommendations for Urban Planning and Policy:
- Update building codes to require the installation of HSWES on all new buildings above 20m height.
- Develop incentives for property owners to install HSWES, such as reduced utility rates and property tax credits.
- Implement policies to ensure a minimum of 20% of rooftops are used for renewable energy installations.
AnyModel: Explore and compare over 50 AI models side-by-side.
Performance Tier
Each model produced high-quality abstracts with accurate technical details, though with varying approaches. ChatGPT 4o delivered a concise, well-structured abstract focused on key metrics. Claude 3.5 Sonnet provided additional context and keywords, enhancing SEO value. Gemini 1.5 Pro created a detailed single-paragraph format with comprehensive technical specifics. Llama 3.1 70B offered the most elaborate response with clear section headings, though perhaps too lengthy for an abstract. Response times varied significantly, with Llama being fastest (2.4s) and Claude slowest (8.3s). For pure abstract writing, ChatGPT 4o's balanced approach was most appropriate.
Budget Tier
Analyzing the responses from all four models reveals distinct performance characteristics. ChatGPT 4o Mini provided a concise, well-structured abstract with key technical details and metrics. Gemini 1.5 Flash delivered a comprehensive response with detailed performance data, though less formally structured. Claude 3.5 Haiku produced the most academically formatted abstract with clear sections and keywords, despite taking longest to generate. Llama 3.1 8B gave the most extensive response, including additional sections beyond a typical abstract, though possibly too detailed for the prompt's intent. All models maintained technical accuracy and included similar core metrics, with processing times ranging from 1.1 to 8.6 seconds.
Sociology Abstract Formatter
Generates sociological research abstracts focusing on social trends and demographic changes. Useful for sociologists and social researchers.
Prompt:
Create a research abstract for this sociology paper examining changing family dynamics in modern society
Example
Performance Results
Abstract
This study explores the evolution of family structures in post-industrial societies from 1960 to 2020, utilizing a mixed-methods approach to assess demographic changes and their societal implications. By analyzing census data from twelve OECD countries and conducting in-depth interviews with individuals representing diverse family arrangements, the research tracks the decline of traditional nuclear family models and the rise of more heterogeneous domestic configurations. Results indicate significant transformations, including a 45% decrease in marriage rates, increased prevalence of single-person households, and a notable rise in cohabitation. The qualitative findings highlight economic influences on family formation, growing acceptance of non-traditional structures, and the impact of technology on familial connections. These transformations suggest profound shifts in social norms and values, affecting social support systems, housing, intergenerational relationships, and policy initiatives. The study underscores the need for adaptive institutional responses to accommodate the evolving landscape of family life in modern societies.
Keywords: family structure, post-industrial society, demographic change, household composition, social evolution
Here's a research abstract for the sociology paper:
Abstract
This study investigates the fundamental transformation of family structures across post-industrial societies between 1960-2020, employing a mixed-methods approach to analyze changing domestic arrangements and their broader societal implications. Using demographic data from twelve OECD nations and qualitative interviews with 50 participants, the research reveals significant shifts in traditional family patterns. Key findings demonstrate a 45% decline in marriage rates, a 115% increase in single-person households, and the emergence of diverse family arrangements including chosen families and cohabiting partnerships. Quantitative analysis of census data shows average household size decreased from 3.6 to 2.3 persons, while qualitative interviews highlight economic factors, technological influence, and evolving social norms as primary drivers of change. The results indicate a clear trend toward more flexible and diverse family structures, suggesting the need for policy adaptations and expanded institutional definitions of family. This research contributes to our understanding of how post-industrial development shapes fundamental social institutions and carries implications for social policy, housing markets, and intergenerational relationships.
Keywords: family structure, post-industrial society, demographic change, household composition, social evolution
This mixed-methods study examines the evolution of family structures in post-industrial societies from 1960 to 2020. Analyzing demographic data from twelve OECD countries alongside qualitative interviews with 50 individuals in diverse family arrangements, we investigate the shift away from the traditional nuclear family model. Quantitative findings reveal significant declines in marriage rates (45%) and average household size (3.6 to 2.3 persons), alongside increases in single-person (13% to 28%) and cohabiting couple households (3% to 15%). Qualitative interviews highlight the influence of economic pressures on delayed family formation, growing acceptance of diverse family structures, the role of technology in maintaining kinship ties, and evolving definitions of family obligations. These findings demonstrate a trend toward smaller, more diverse households, reflecting broader societal shifts in values and economic conditions. This diversification has significant implications for social support systems, housing markets, intergenerational relationships, and work-life balance policies, necessitating a re-evaluation of traditional family definitions within institutional contexts. This research was supported by the National Science Foundation, Grant #2021-0789.
Keywords: family structure, post-industrial society, demographic change, household composition, social evolution, mixed-methods
Abstract:
Title: The Evolution of Family Structures in Post-Industrial Societies: A Mixed-Methods Analysis
This study examines the transformation of family structures in post-industrial societies between 1960-2020, employing a mixed-methods approach to investigate demographic trends and qualitative interviews with diverse family arrangements. The findings reveal a significant shift towards smaller, more diverse household arrangements, driven by broader socioeconomic and cultural changes. The research highlights the implications of these changes for social support systems, housing markets, intergenerational relationships, and work-life balance policies, underscoring the need for policy adaptations and expanded definitions of family in institutional contexts.
Keywords: family structure, post-industrial society, demographic change, household composition, social evolution
AnyModel: Explore and compare over 50 AI models side-by-side.
Budget Results
Abstract
This research investigates the transformation of family structures in post-industrial societies between 1960 and 2020, employing a mixed-methods approach that combines quantitative demographic trends with qualitative insights from diverse participants. The study elucidates the shifting dynamics from the traditional nuclear family model to a more pluralistic array of family arrangements, prompted by socioeconomic changes, evolving cultural norms, and technological advancements. Quantitative data derived from national censuses across twelve OECD countries reveals significant trends: a 45% decline in marriage rates, a climb in single-person households from 13% to 28%, a drop in average household size from 3.6 to 2.3 persons, and an increase in cohabiting couples from 3% to 15%. Complementing the quantitative analysis, semi-structured interviews with 50 individuals aged 25-65 expose critical themes including economic pressures leading to delayed family formation, growing societal acceptance of varied family structures, and the impact of technology on family relationships. The findings illuminate the structural changes in family dynamics and their broader societal implications, such as the necessity for adaptable social support systems, redefined housing policies, and a reconsideration of intergenerational connections. Conclusively, the evolution towards more diverse and flexible family units underscores the need for progressive policy approaches and a broader conceptualization of family within institutional frameworks.
Keywords: family structure, post-industrial society, demographic change, household composition, social evolution.
The Evolution of Family Structures in Post-Industrial Societies: A Mixed-Methods Analysis
This study examines the dramatic transformation of family structures in twelve OECD countries from 1960 to 2020, employing a mixed-methods approach combining quantitative demographic analysis with qualitative interviews. Quantitative data from national censuses reveal significant declines in marriage rates (45%), average household size (from 3.6 to 2.3 persons), and a corresponding rise in single-person households (from 13% to 28%) and cohabiting couples (from 3% to 15%). Qualitative data, gathered through semi-structured interviews with 50 participants (aged 25-65) representing diverse family arrangements, explored the lived experiences shaping these trends. Key themes emerging from the interviews included the influence of economic factors on delayed family formation, growing societal acceptance of diverse family structures, the role of technology in maintaining familial connections, and evolving conceptions of family obligations. The findings demonstrate a clear shift toward smaller, more diverse household arrangements, reflecting broader societal changes in values and economic conditions. These evolving family structures have profound implications for social support systems, housing markets, intergenerational relationships, and the need for adaptable work-life balance policies. This research highlights the necessity of revising institutional definitions of "family" to reflect the increasing diversity and fluidity of contemporary family life. This work was supported by the National Science Foundation, Grant #2021-0789.
Abstract
This study investigates the transformation of family structures in post-industrial societies from 1960-2020, employing a mixed-methods approach to examine demographic trends and individual experiences. Through quantitative analysis of census data and qualitative interviews, the research reveals significant shifts in household composition, challenging traditional nuclear family models. Key findings demonstrate a marked decline in marriage rates, increased single-person households, and emerging diverse family arrangements. The study highlights how economic, technological, and cultural factors contribute to evolving domestic landscapes, suggesting profound implications for social institutions and policy frameworks.
Key Findings
- 45% decline in marriage rates
- 28% of households now single-person
- Cohabiting couples increased to 15%
- Average household size reduced to 2.3 persons
Significance
The research provides a comprehensive exploration of family dynamics, illuminating the complex interplay between societal changes and personal family experiences in contemporary post-industrial contexts.
Keywords: family structure, demographic change, post-industrial society, household composition
Funding: National Science Foundation, Grant #2021-0789
Here's an abstract that highlights the research while maintaining academic integrity:
Title: The Evolution of Family Structures in Post-Industrial Societies: A Mixed-Methods Analysis
Abstract:
The traditional nuclear family model is undergoing significant changes in post-industrial societies. This study examines the transformation of family structures in post-industrial societies from 1960-2020, using a mixed-methods approach that incorporates both quantitative demographic analysis and qualitative interviews. Our data reveals a clear trend toward smaller, more diverse household arrangements, reflecting broader societal shifts in values and economic conditions. Specifically, we found that marriage rates declined by 45% between 1960-2020, single-person households increased from 13% to 28%, and cohabiting couples rose from 3% to 15% of households. The qualitative component of this study highlighted the importance of economic factors, increased acceptance of alternative family structures, and technology's role in maintaining family connections. Our findings have significant implications for social support systems, housing markets, intergenerational relationships, and work-life balance policies. This research concludes that family structures in post-industrial societies continue to evolve toward greater diversity and flexibility, necessitating policy adaptations and expanded definitions of family in institutional contexts.
Keywords: family structure, post-industrial society, demographic change, household composition, social evolution
AnyModel: Explore and compare over 50 AI models side-by-side.
Performance Tier
ChatGPT 4.0 provided comprehensive detail while maintaining clarity, effectively balancing quantitative data with qualitative insights. Claude 3.5 Sonnet offered similar depth but with more specific statistical details and structured formatting. Gemini 1.5 Pro's response was the most detailed, including grant information and broader statistical ranges, though took longest to generate. Llama 3.1 70B provided the most concise response, delivering key points efficiently in under 1 second, but lacked specific statistical data. All models maintained appropriate academic tone and included relevant keywords, with Gemini adding an extra keyword. For detailed research abstracts, Gemini and ChatGPT showed strongest performance, while Llama excelled at quick, succinct summaries.
Budget Tier
In this comparison of AI models generating sociology paper abstracts, each model demonstrated distinct capabilities. ChatGPT 4o Mini provided a well-structured abstract with comprehensive quantitative data and clear thematic organization. Gemini 1.5 Flash offered similar depth but included additional grant information and more detailed policy implications. Claude 3.5 Haiku uniquely formatted its response with clear sections and bullet points, making it more scannable. Llama 3.1 8B, despite being the fastest (665ms), delivered a cohesive abstract but with less sophisticated organization. All models maintained consistent statistical data, though their presentation styles varied significantly. Claude demonstrated the best balance of content organization and academic formatting.
Computer Science Abstract Creator
Creates technical abstracts for computer science research, emphasizing algorithmic approaches and results. Essential for IT professionals and researchers.
Prompt:
Write an abstract for this computer science paper on machine learning applications in cybersecurity
Example
Performance Results
This paper presents a comprehensive evaluation of machine learning approaches for detecting network security threats, highlighting their efficacy in real-time anomaly detection and threat identification. We explore various classification algorithms, including Random Forest, Support Vector Machines, Deep Neural Networks, Gradient Boosting, and K-Nearest Neighbors, assessing their performance based on a dataset of 1 million network flows characterized by features such as protocol type, service, and payload entropy. Through meticulous preprocessing and feature engineering, the models were trained and optimized to achieve high accuracy and low false positive rates. The Random Forest model demonstrated superior effectiveness with an accuracy of 97.8% and an F1-score of 96.2%, while Deep Neural Networks, despite requiring more computational resources, also performed robustly. This study underscores machine learning's potential in enhancing network security by automating and refining threat detection mechanisms. We also discuss the limitations concerning model adaptability and propose future research directions focusing on real-time implementation and adversarial resilience to bolster cybersecurity defenses.
Here's a polished abstract for the paper:
Abstract
This research presents an innovative machine learning framework for detecting network security threats in real-time environments. We developed and evaluated a comprehensive system utilizing multiple classification algorithms to identify malicious network behavior, with particular focus on minimizing false positives while maintaining high detection rates. Our methodology encompassed the analysis of 1 million network flows, incorporating both benign and malicious traffic patterns, with features extracted from packet headers and payload data. The study compared five machine learning approaches: Random Forest, Support Vector Machines, Deep Neural Networks, Gradient Boosting, and K-Nearest Neighbors. Results demonstrate that Random Forest achieved superior performance with 97.8% accuracy and 96.2% F1-score, significantly outperforming traditional detection methods. The framework shows particular promise in identifying sophisticated attack patterns while maintaining computational efficiency. Our findings suggest that machine learning-based approaches can provide robust, adaptive security solutions for modern network environments, though challenges remain in areas of model maintenance and processing overhead. This work contributes to the growing body of research in automated cybersecurity defense systems and provides a foundation for future developments in real-time threat detection.
Keywords: network security, machine learning, threat detection, classification algorithms, cybersecurity
This paper explores the application of machine learning for network security threat detection. A comprehensive framework is presented, evaluating several classification algorithms including Random Forest, Support Vector Machines, Deep Neural Networks, Gradient Boosting, and K-Nearest Neighbors. The models are trained and tested on a dataset of 1 million network flows, featuring extracted characteristics such as protocol type, service, connection duration, and payload entropy. Performance is assessed using metrics like accuracy, precision, recall, F1-score, AUC, false positive rate, and detection latency. Results demonstrate the effectiveness of these methods, with Random Forest achieving the highest accuracy (97.8%) and F1-score (96.2%). The paper discusses the relative strengths and limitations of each model, highlighting the trade-off between accuracy and computational resources. Future work will focus on real-time implementation, feature selection optimization, and enhancing robustness against adversarial attacks.
Here is the rewritten abstract:
Title: Machine Learning Approaches for Network Security Threat Detection
Abstract: The rapid evolution of network security threats necessitates advanced detection methods. This paper presents a comprehensive framework for detecting network security threats using machine learning algorithms. We evaluate the effectiveness of multiple classification methods, including Random Forest, Support Vector Machines, Deep Neural Networks, Gradient Boosting, and K-Nearest Neighbors, in identifying malicious network behavior. Our results demonstrate that machine learning algorithms can achieve high accuracy and low false positive rates, with Random Forest achieving the best overall performance (97.8% accuracy, 96.2% F1-score). This work highlights the potential of machine learning in real-time network traffic threat detection and paves the way for further research in optimizing feature selection, handling adversarial attacks, and implementing transfer learning approaches. Our findings contribute to the advancement of network security threat detection and provide a foundation for future studies in cybersecurity.
AnyModel: Explore and compare over 50 AI models side-by-side.
Budget Results
Abstract:
In this paper, we propose a robust framework that leverages machine learning algorithms for the detection of network security threats. With the increasing sophistication of cyber-attacks, traditional detection methods are becoming insufficient, motivating our exploration of advanced techniques. We evaluate a diverse set of classification models, including Random Forest, Support Vector Machines, Deep Neural Networks, Gradient Boosting, and K-Nearest Neighbors, using a dataset of 1 million network flows composed of both benign and malicious traffic. Our methodology encompasses comprehensive data collection through tcpdump, extensive feature engineering involving protocol characteristics and flow metrics, and meticulous preprocessing to enhance model performance. Our results reveal that the Random Forest model exhibits the highest accuracy at 97.8%, alongside impressive precision and recall metrics, outpacing other evaluated models. We discuss the implications of our findings, addressing the advantages and limitations of each approach, including the constraints of model retraining and processing overhead. Future work emphasizes the need for real-time implementation, feature optimization, and resilience against adversarial attacks. This study reinforces the efficacy of machine learning in enhancing cybersecurity protocols, providing a foundation for ongoing advancements in threat detection methodologies.
Keywords: network security, machine learning, threat detection, classification algorithms, cybersecurity
This paper investigates the application of machine learning for network security threat detection. A comprehensive framework is presented, evaluating Random Forest, Support Vector Machines, Deep Neural Networks, Gradient Boosting, and K-Nearest Neighbors on a dataset of 1 million network flows. The models were trained and evaluated using a 70/30 train-test split, 5-fold cross-validation, and hyperparameter optimization via grid search. Random Forest achieved the highest accuracy (97.8%), precision (96.5%), recall (95.9%), and F1-score (96.2%), outperforming other models, although Deep Neural Networks required significantly more computational resources. While demonstrating effective threat detection, the study also identifies limitations including model retraining needs, processing overhead, and false positive management. Future work focuses on real-time implementation, optimized feature selection, adversarial attack resistance, and the exploration of transfer learning techniques.
Here's a professional abstract for the paper:
Abstract
Network security threats are becoming increasingly complex, requiring sophisticated detection mechanisms that can adapt to evolving attack strategies. This research presents a comprehensive machine learning framework for identifying and classifying network security threats using advanced classification algorithms. By leveraging a diverse dataset of 1 million network flows, we systematically evaluated multiple machine learning models to assess their effectiveness in detecting malicious network behavior.
Our experimental methodology involved rigorous feature engineering, preprocessing, and model training across five different machine learning algorithms: Random Forest, Support Vector Machines, Deep Neural Networks, Gradient Boosting, and K-Nearest Neighbors. The performance evaluation considered critical metrics including accuracy, precision, recall, F1-score, and false positive rate.
The results demonstrate that the Random Forest algorithm achieved the highest performance, with an impressive 97.8% accuracy and 96.5% precision in threat detection. Deep Neural Networks and other models also showed robust capabilities, highlighting the potential of machine learning techniques in cybersecurity threat identification. Our findings contribute to the growing body of research on adaptive, intelligent network security solutions and provide insights into the comparative strengths of different machine learning approaches.
Keywords: network security, machine learning, threat detection, classification algorithms, cybersecurity
Title: Machine Learning Approaches for Network Security Threat Detection
Abstract:
This paper presents a comprehensive framework for detecting network security threats using machine learning algorithms. By leveraging a large dataset of network flows, we evaluate and compare the effectiveness of various classification methods, including Random Forest, Support Vector Machines, Deep Neural Networks, Gradient Boosting, and K-Nearest Neighbors. Our results demonstrate that Random Forest achieved the best overall performance, with a high accuracy of 97.8% and low false positive rates. We highlight the strengths and weaknesses of each model, including computational requirements and performance on specific attack types. Our study contributes to the development of robust and efficient network security solutions, providing a framework for future research and implementation in real-world scenarios.
AnyModel: Explore and compare over 50 AI models side-by-side.
Performance Tier
The comparison reveals distinct performance patterns across models. ChatGPT 4.0 produced a well-structured, technically precise abstract focusing on methodology and results. Claude 3.5 Sonnet added formatting elements and keywords but maintained similar content. Gemini 1.5 Pro delivered a concise, data-focused version emphasizing performance metrics. Llama 3.1 70B was notably faster (1103ms vs 4000-5500ms for others) and provided a clear, academic-style abstract with a strong opening context. While all models captured the key information, ChatGPT and Claude showed better natural language flow, while Gemini and Llama excelled in technical precision and efficiency.
Budget Tier
Analyzing the four AI models' responses to the cybersecurity paper abstract prompt reveals distinct performance characteristics. ChatGPT 4o Mini produced a detailed, well-structured abstract but took longest at 9299ms. Claude 3.5 Haiku generated the most comprehensive and formally structured response in 7068ms. Gemini 1.5 Flash delivered a concise yet complete abstract with specific metrics in 7864ms. Llama 3.1 8B was notably fastest at 477ms with a shorter but well-organized abstract, though lacking some technical depth compared to others. All models captured the key information about Random Forest's 97.8% accuracy, but Claude and ChatGPT provided superior academic formatting and keyword implementation.
Biology Research Abstract Generator
Produces scientific abstracts for biological research, highlighting experimental methods and findings. Useful for biologists and environmental scientists.
Prompt:
Generate a formal abstract for this biological study on plant adaptation to climate change
Example
Performance Results
Abstract:
This study explores the adaptive responses of three native plant species (Artemisia californica, Salvia leucophylla, and Baccharis pilularis) to climate-induced environmental stress through a comprehensive investigation of their physiological and morphological changes. The research, conducted over a 24-month period, utilizes a controlled experimental design with 150 specimens per species exposed to elevated temperatures and reduced precipitation, in addition to control conditions. Key adaptive mechanisms identified include significant increases in leaf thickness under elevated temperatures and reductions in stomatal density under decreased precipitation, indicating enhanced drought tolerance. Phenological shifts were observed with earlier flowering times, suggesting a strategic avoidance of peak stress periods. Enhanced water use efficiency was indicated by altered carbon isotope ratios, while increased root:shoot ratios highlighted improved resource acquisition strategies. These findings collectively underscore the capacity of native species to adapt to projected climatic changes through multifaceted modifications that enhance their survival potential. Future research should focus on the long-term fitness implications of these adaptations in native plant populations.
Abstract
This comprehensive study examines adaptive responses of three California native plant species (Artemisia californica, Salvia leucophylla, and Baccharis pilularis) to simulated climate change conditions over a 24-month period. Through controlled experiments involving 450 total specimens, we investigated morphological, physiological, and phenological adaptations under elevated temperature (+2°C) and reduced precipitation (-30%) treatments.
Results revealed significant morphological modifications across all species, including increased leaf thickness (12.7-18.3%, p<0.001) and decreased stomatal density (19.8-25.2%, p<0.001) under stress conditions. Phenological adaptations manifested as advanced flowering times (9.7-14.2 days earlier), while physiological changes included improved water use efficiency (δ13C differences of -1.6 to -2.1‰) and increased root:shoot ratios (19.8-24.6%).
These findings demonstrate substantial adaptive plasticity in native plant species facing climate stress, suggesting potential resilience to projected environmental changes. However, further research is needed to evaluate long-term fitness implications of these adaptive responses.
Keywords: climate adaptation, plant physiology, phenological plasticity, drought tolerance, native species
This study investigated the adaptive capacity of three native California plant species (Artemisia californica, Salvia leucophylla, and Baccharis pilularis) to simulated climate change conditions. Over a 24-month period (January 2020 - December 2021), 150 specimens per species were exposed to control, elevated temperature (+2°C), and reduced precipitation (-30%) treatments. Morphological adaptations included significant increases in leaf thickness (12.7-18.3%) under elevated temperatures and significant decreases in stomatal density (19.8-25.2%) under reduced precipitation across all species. Physiological responses included improved water use efficiency, as indicated by decreased δ13C values (1.6-2.1‰ difference between control and treatment groups), and increased root:shoot ratios (19.8-24.6%) under stress conditions. Phenological adaptation was observed through advanced flowering times (9.7-14.2 days). These results demonstrate a suite of adaptive mechanisms employed by these species to mitigate the effects of climate-induced stress, including morphological adjustments for enhanced drought tolerance, physiological changes for improved resource acquisition, and phenological shifts to avoid periods of peak stress. While these adaptations suggest potential resilience to projected climate change, long-term fitness consequences warrant further investigation. This research was supported by the National Science Foundation (Grant #NSF-2021-0789) and the California Native Plant Society.
Here's a rewritten, polished formal abstract for the biological study:
Title: Adaptive Mechanisms of Native Plant Species in Response to Climate-Induced Environmental Stress
Abstract: Climate change poses significant threats to plant species worldwide, necessitating a deeper understanding of adaptive mechanisms underlying their responses to environmental stress. This study investigates the physiological and morphological adaptations of three native plant species (Artemisia californica, Salvia leucophylla, and Baccharis pilularis) under simulated climate change conditions. Our 24-month experiment revealed significant modifications in leaf structure, water retention, and reproductive timing across populations exposed to elevated temperatures and altered precipitation patterns. The results demonstrate enhanced drought tolerance through morphological adaptations, such as increased leaf thickness and decreased stomatal density, as well as phenological shifts in flowering initiation. Furthermore, improved water use efficiency and enhanced root development suggest that these native plant species can adapt to projected climate conditions. This study highlights the complex and multifaceted nature of plant adaptation to climate stress and underscores the need for continued research into the long-term fitness consequences of these adaptations.
AnyModel: Explore and compare over 50 AI models side-by-side.
Budget Results
Title: Adaptive Mechanisms of Native Plant Species in Response to Climate-Induced Environmental Stress
Authors: Sarah Chen, Michael Rodriguez, Elena Popov
Department of Plant Biology, University of California
Abstract:
This study explores the adaptive mechanisms employed by three native plant species—Artemisia californica, Salvia leucophylla, and Baccharis pilularis—amid simulated climate change scenarios. Conducted over a 24-month period, the research examined physiological and morphological adaptations in response to elevated temperatures and reduced precipitation. We established an experimental framework consisting of 150 specimens per species, divided into control and treatment groups exposed to a +2°C temperature increase and a -30% precipitation reduction. Key metrics, including leaf thickness, stomatal density, water use efficiency, flowering time, seed production, and root development, were measured.
Results indicated significant morphological adaptations: leaf thickness increased across all species under elevated temperatures (A. californica: +18.3%, S. leucophylla: +12.7%, B. pilularis: +15.9%; p<0.001 for all), while stomatal density decreased in response to reduced precipitation. Flowering initiation increased by an average of 12.3 days in A. californica, 9.7 days in S. leucophylla, and 14.2 days in B. pilularis. Enhanced water use efficiency was inferred from carbon isotope ratios (δ13C), showing mean differences of -1.8‰, -2.1‰, and -1.6‰, respectively. Additionally, root:shoot ratios improved under stress, with increases of +24.6% in A. californica, +19.8% in S. leucophylla, and +22.3% in B. pilularis.
These findings elucidate significant adaptive responses, positioning morphological traits such as increased leaf thickness and reduced stomatal density as critical factors in drought resilience. The advanced flowering timelines reflect a strategic phenological shift to mitigate stress impacts. Furthermore, the enhanced root systems highlight effective resource acquisition strategies. In conclusion, this research underscores the capacity of native plant species to adapt morphologically, physiologically, and phenologically to climate-induced environmental stressors, suggesting potential pathways for enhancing survival under future climate scenarios. Future research should evaluate the long-term fitness implications of these adaptations.
Keywords: climate change, native plants, adaptation, environmental stress, physiological ecology, phenological changes.
Adaptive Mechanisms of Native Californian Plant Species in Response to Simulated Climate Change
Sarah Chen, Michael Rodriguez, Elena Popov Department of Plant Biology, University of California
This study investigated the physiological and morphological adaptations of three native Californian plant species, Artemisia californica, Salvia leucophylla, and Baccharis pilularis, to simulated climate change conditions. Over a 24-month period, 150 individuals of each species were subjected to control, elevated temperature (+2°C), and reduced precipitation (-30%) treatments. Measurements of leaf thickness, stomatal density, water use efficiency (via δ¹³C analysis), flowering time, seed production, and root development were conducted. All three species exhibited significant adaptive responses. Elevated temperatures induced significant increases in leaf thickness (12.7-18.3%, p<0.001 across species), while reduced precipitation led to significant decreases in stomatal density (19.8-25.2%, p<0.001). Flowering time advanced significantly across all species (9.7-14.2 days), and water use efficiency improved under both stress treatments (mean δ¹³C difference: -1.6 to -2.1‰). Finally, root:shoot ratios increased significantly under both stress treatments (19.8-24.6%). These findings suggest that these native species possess considerable adaptive capacity to cope with projected climate change impacts, though further research is needed to assess long-term fitness consequences. This research was supported by the National Science Foundation (Grant #NSF-2021-0789) and the California Native Plant Society.
Formal Abstract: Adaptive Mechanisms of Native Plant Species in Response to Climate-Induced Environmental Stress
Abstract
Objective: This study investigated physiological and morphological adaptations of three native California plant species to simulated climate change conditions.
Methods: A 24-month experimental design examined Artemisia californica, Salvia leucophylla, and Baccharis pilularis under controlled temperature (+2°C) and precipitation (-30%) stress conditions. 150 specimens per species were analyzed across morphological, physiological, and phenological parameters.
Key Findings:
- Significant leaf thickness increases (12.7-18.3%) across all species
- Substantial stomatal density reductions (19.8-25.2%) under water stress
- Accelerated flowering initiation (9.7-14.2 days earlier)
- Improved water use efficiency demonstrated through carbon isotope ratios
- Enhanced root:shoot ratios (19.8-24.6%) under stress conditions
Conclusions: Native plant species demonstrate multiple adaptive mechanisms, including morphological restructuring and phenological shifts, suggesting potential resilience to climate change-induced environmental stress.
Significance: Provides critical insights into native plant adaptation strategies, informing conservation and ecological management approaches in increasingly variable climatic conditions.
Keywords: Climate adaptation, plant physiology, environmental stress, native species, California flora
Title: Adaptive Mechanisms of Native Plant Species in Response to Climate-Induced Environmental Stress
Abstract:
Climate change poses a significant threat to native plant species worldwide, necessitating a comprehensive understanding of their adaptability to projected environmental stressors. This study investigated the physiological and morphological adaptations of three native plant species (Artemisia californica, Salvia leucophylla, and Baccharis pilularis) in response to simulated climate change conditions, characterized by elevated temperatures (+2°C) and altered precipitation patterns (-30%). Over a 24-month monitoring period, we observed pronounced adaptive responses across all studied species, including:
- Morphological adaptations: increases in leaf thickness and decreases in stomatal density indicating enhanced drought tolerance.
- Phenological changes: advancement of flowering initiation, suggesting an adaptive shift to avoid peak stress periods.
- Water use efficiency: improved carbon isotope ratios (δ13C) indicating enhanced resource conservation.
- Root development: increased root:shoot ratios pointing to improved resource acquisition strategies under stress conditions.
These findings demonstrate the capacity of native plant species to exhibit multiple adaptive mechanisms in response to climate stress, potentially enhancing their survival capacity under projected climate conditions. While the long-term fitness consequences of these adaptations require further investigation, our results contribute valuable insights into the resilience of native plant populations and inform conservation strategies aimed at mitigating the impacts of climate change.
Authors: Sarah Chen, Michael Rodriguez, Elena Popov Department of Plant Biology, University of California
AnyModel: Explore and compare over 50 AI models side-by-side.
Performance Tier
The models showed varying strengths in generating a formal scientific abstract. ChatGPT 4o produced a well-structured abstract with clear methodology and findings, though less specific in numerical data. Claude 3.5 Sonnet excelled with precise statistical details and proper scientific formatting, including keywords. Gemini 1.5 Pro provided the most comprehensive response, including grant information and detailed measurements, but was less concise. Llama 3.1 70B was notably faster (1246ms) and produced a polished abstract with good scientific tone, though lacking specific numerical data. Claude appeared most balanced in terms of structure, detail, and scientific rigor.
Budget Tier
ChatGPT 4o Mini generated a comprehensive abstract with detailed methodology and results, but was verbose at 400+ words. Gemini 1.5 Flash produced a more concise version with similar technical depth. Claude 3.5 Haiku created the most efficiently structured abstract using clear section headers and bullet points, making it highly readable while maintaining key information. Llama 3.1 8B delivered a well-organized abstract with numbered points, though slightly less detailed in the results section. Claude demonstrated superior formatting, while Gemini excelled at data precision. Response times varied significantly, with Llama being notably faster at 689ms versus Gemini's 15006ms.
Literature Review Abstract Creator
Creates humanities-style abstracts for literature reviews and theoretical analyses. Valuable for literature scholars and cultural theorists.
Prompt:
Compose an abstract for this literature review on the evolution of feminist theory in 20th century writing
Example
Performance Results
This literature review traces the evolution of feminist literary theory through the twentieth century, capturing its development across various waves and frameworks. From the foundational insights of Virginia Woolf in "A Room of One's Own" regarding women's literary expression and economic independence, to Simone de Beauvoir’s "The Second Sex" introducing the concept of woman as "Other," early feminist criticism laid crucial groundwork for understanding gender dynamics in literature. The emergence of gynocriticism in the 1960s and 1970s, led by Elaine Showalter, sought to recover and establish a women's literary tradition, while political feminist criticism, exemplified by Kate Millett's "Sexual Politics," scrutinized how texts perpetuate patriarchal ideologies.
French feminist theory introduced radical concepts like écriture féminine through Hélène Cixous and further explored gender and language with figures such as Julia Kristeva and Luce Irigaray. The 1980s brought psychoanalytic approaches from scholars like Gilbert and Gubar, whose "The Madwoman in the Attic" analyzed women's literature through psychological lenses. Poststructuralist feminism, as articulated in Judith Butler's "Gender Trouble," challenged essentialist views of gender and emphasized performativity.
During the 1990s, intersectional feminist criticism became prominent, with scholars like bell hooks and Gloria Anzaldúa examining the interplay of gender with race, class, and sexuality. Patricia Hill Collins's "Black Feminist Thought" highlighted literature's multifaceted oppression portrayals, reflecting an evolved understanding of gender complexity.
This comprehensive development mirrored broader societal shifts and intellectual currents, transitioning from representation and recovery to nuanced analyses of language, power, and identity. By 2000, feminist literary theory had cemented itself as a dynamic and multifaceted discipline, vital to literary studies for challenging canonical norms and deconstructing gender representation, continuing to inform contemporary literary and cultural critique.
Evolution of Feminist Literary Theory: A Century of Development (1900-2000)
This comprehensive review examines the transformation of feminist literary theory throughout the twentieth century, tracking its development from early foundational works to complex contemporary frameworks. The evolution spans from Virginia Woolf's seminal exploration of gender and creativity to intersectional approaches addressing multiple forms of oppression in literature.
Key Developments:
- Establishment of foundational concepts through Woolf's "A Room of One's Own" (1929)
- Introduction of woman as "Other" by de Beauvoir (1949)
- Development of gynocriticism in the 1970s
- Emergence of French feminist theory and écriture féminine
- Integration of psychoanalytic and poststructuralist approaches
- Rise of intersectional analysis in the 1990s
The field progressed from initial focus on representation and recovery to sophisticated analyses of language, power, and identity. By 2000, feminist literary theory had developed into a multifaceted discipline incorporating diverse theoretical perspectives and methodological approaches, establishing itself as an essential component of literary studies.
Major Theoretical Frameworks:
- Anglo-American feminist criticism
- French feminist theory
- Gynocriticism
- Psychoanalytic feminist criticism
- Poststructuralist feminism
- Intersectional feminist criticism
This evolution reflects broader social movements and intellectual developments, demonstrating the field's continuing influence on contemporary literary analysis and cultural criticism.
This literature review examines the evolution of feminist literary theory throughout the 20th century, tracing its development through distinct waves and theoretical frameworks. Beginning with Virginia Woolf's foundational work on women's writing and economic independence, the review explores key contributions such as Simone de Beauvoir's concept of "Otherness," Elaine Showalter's gynocriticism, and Kate Millett's political feminist critique. The emergence of French feminist theory, including the work of Hélène Cixous, Julia Kristeva, and Luce Irigaray, is analyzed alongside the rise of psychoanalytic approaches by Sandra Gilbert and Susan Gubar. The review also considers the impact of poststructuralism, exemplified by Judith Butler's performativity theory, and the growing importance of intersectional analyses spearheaded by bell hooks, Gloria Anzaldúa, and Patricia Hill Collins. By charting the progression from initial concerns with representation and recovery to complex analyses of language, power, and identity, this review demonstrates how feminist literary theory, encompassing diverse methodologies and influential texts, became a multifaceted and vital field within literary studies by the end of the 20th century.
Title: A Century of Evolution: 20th-Century Feminist Literary Theory and Its Shaping of Literary Studies
Abstract:
This literature review charts the development of feminist literary theory throughout the 20th century, tracing its emergence and evolution through multiple waves and theoretical frameworks. From Virginia Woolf's foundational "A Room of One's Own" (1929) to the intersectional approaches of the 1990s, feminist literary theory expanded to encompass diverse methodological approaches and perspectives. Major frameworks, including Anglo-American feminist criticism, French feminist theory, and poststructuralist feminism, intersected and influenced one another, shaping the discipline into a complex, multifaceted field. Influential texts, such as "The Yellow Wallpaper" (1892) and "The Color Purple" (1982), both exemplified and contributed to evolving theoretical approaches to gender and literature. This review demonstrates how feminist literary theory mirrored broader social movements and intellectual developments, moving from concerns with representation and recovery to sophisticated analyses of language, power, and identity. By 2000, feminist literary theory had solidified its position as an essential component of literary studies, providing essential tools for analyzing texts, challenging canonical assumptions, and understanding literature's role in shaping gender construction and representation.
AnyModel: Explore and compare over 50 AI models side-by-side.
Budget Results
Abstract:
This literature review examines the evolution of feminist literary theory throughout the twentieth century, tracing its development across significant theoretical frameworks and social movements. Beginning with Virginia Woolf's seminal work, "A Room of One's Own" (1929), which laid the groundwork for discussions on women's literary expression and economic independence, the review explores critical contributions from influential theorists. Simone de Beauvoir's "The Second Sex" (1949) introduced foundational concepts of female marginalization, while the rise of gynocriticism in the 1960s and 1970s, as articulated by Elaine Showalter, focused on recovering women's narratives. The emergence of French feminist theory, spearheaded by figures like Hélène Cixous and Julia Kristeva, introduced new perspectives on language and identity. The review further highlights the integration of psychoanalytic approaches and poststructuralist critiques, exemplified by the works of Gilbert and Gubar, as well as Judith Butler's exploration of gender performativity. As the century progressed, intersectional frameworks gained prominence, reflecting a nuanced understanding of how race, class, and sexuality intersect with gender in literary texts. By 2000, feminist literary theory had established itself as a multifaceted discipline, offering diverse methods for analyzing literature and challenging traditional canonization. This review underscores the field's ongoing relevance in contemporary literary studies, illustrating how its theoretical advancements resonate within current cultural criticism and literary analysis.
This literature review traces the evolution of feminist literary theory throughout the 20th century, charting its development from foundational concerns with women's representation and the recovery of lost female voices to sophisticated analyses of language, power, and identity. Beginning with early explorations of gender constraints in writing, as exemplified by Virginia Woolf's work, the review examines the key shifts in feminist literary criticism, including the emergence of gynocriticism, French feminist theory, psychoanalytic approaches, poststructuralism, and intersectionality. Key figures such as Simone de Beauvoir, Elaine Showalter, Hélène Cixous, Julia Kristeva, Judith Butler, and bell hooks are highlighted, showcasing the diverse theoretical frameworks that shaped the field. The review demonstrates how these evolving theoretical perspectives, informed by broader social and intellectual movements, enriched the understanding of gender's role in literary production, authorship, and interpretation, ultimately establishing feminist literary theory as a crucial and multifaceted component of literary studies by the year 2000.
Here's an abstract for the literature review:
Abstract
This comprehensive review examines the evolution of feminist literary theory throughout the twentieth century, tracing its development from foundational critiques to sophisticated intersectional analyses. Beginning with Virginia Woolf's seminal work in the 1920s and progressing through multiple theoretical waves, the study demonstrates how feminist literary criticism transformed from marginalized discourse to a complex, multidisciplinary field of scholarly inquiry.
The review identifies key theoretical shifts, including the emergence of gynocriticism, French feminist theory, psychoanalytic approaches, and intersectional methodologies. By analyzing pivotal texts and scholars such as Simone de Beauvoir, Elaine Showalter, Hélène Cixous, and Judith Butler, the research illuminates the progressive sophistication of feminist literary perspectives.
Central themes include the deconstruction of patriarchal literary structures, recovery of women's literary traditions, exploration of gender performativity, and examination of intersectional identities. The study argues that feminist literary theory fundamentally reshaped understanding of textual representation, authorship, and critical interpretation by the century's end.
Keywords: Feminist literary theory, gender studies, literary criticism, intersectionality, twentieth-century literature
Abstract:
"Feminist Literary Theory: A Century of Development (1900-2000)" is a comprehensive literature review tracing the emergence, evolution, and diversification of feminist theory in 20th-century writing. From Virginia Woolf's foundational concepts on women's literary expression to the sophisticated analyses of intersectionality and performativity, this review showcases the significant shifts and advancements in feminist literary theory. Spanning over a century, the review surveys major waves of feminist thought, including Anglo-American feminist criticism, French feminist theory, gynocriticism, psychoanalytic feminist criticism, poststructuralist feminism, and intersectional feminist criticism. Through an examination of influential texts and theoretical frameworks, the review illustrates how feminist literary theory has matured into a complex discipline, equipping scholars with diverse tools to analyze texts, challenge canonical assumptions, and understand literature's role in shaping and reflecting social norms and power structures. By 2000, feminist literary theory had become an indispensable component of literary studies, shaped by changing social contexts and intellectual paradigms. The review provides a critical historical overview of the theoretical evolution, demonstrating the ongoing relevance and impact of feminist literary theory on contemporary literary analysis and cultural criticism.
AnyModel: Explore and compare over 50 AI models side-by-side.
Performance Tier
ChatGPT 4.0 and Claude 3.5 provided the most comprehensive analyses, with Claude offering better structure through clear headings and bullet points. Gemini 1.5 Pro delivered a more concise but still thorough response in a single paragraph. Llama 3.1 70B was notably faster (1450ms vs 5000-6000ms for others) and produced a well-organized abstract with good historical context. All models accurately captured key theorists and movements, but Claude's structured format made information more accessible. ChatGPT offered the most detailed theoretical progression, while Llama excelled at concision without sacrificing essential content. Gemini struck a good balance between detail and brevity.
Budget Tier
All models demonstrated strong comprehension of feminist literary theory evolution, producing coherent abstracts with key theorists and movements. ChatGPT 4o Mini delivered a well-structured response highlighting major developments chronologically. Gemini 1.5 Flash offered a more concise summary while maintaining core concepts. Claude 3.5 Haiku uniquely included formal academic elements like keywords and section headers. Llama 3.1 8B was notably efficient, completing the task in just 659ms while providing comprehensive coverage. Claude's response was most academically formatted, while Llama's showed the best balance of depth and processing speed.
Physics Research Abstract Generator
Generates technical abstracts for physics research, focusing on methodology and experimental results. Essential for physicists and quantum computing researchers.
Prompt:
Create a scientific abstract for this physics paper on quantum computing advancements
Example
Performance Results
In this paper, we report on significant advancements in the architecture of scalable quantum computing systems utilizing superconducting transmon qubits. Our research introduces an innovative method to scale quantum processors while preserving coherence times and gate fidelities, key metrics for effective quantum computation. Employing a 20-qubit processor configured in a two-dimensional lattice, we achieved enhanced multi-qubit coupling schemes and reduced crosstalk, critical for operating larger quantum systems. Through the use of tunable couplers based on superconducting quantum interference devices (SQUIDs), we realized precise control over qubit interactions, achieving average two-qubit gate fidelities of 99.2% and single-qubit gate fidelities of 99.8%.
Our experimental setup features qubit frequencies ranging from 4.5-5.5 GHz, with relaxation (T1) and coherence (T2) times recorded at 50-70 μs and 40-60 μs, respectively. Through dynamic control of inter-qubit coupling strengths, tunable from -20 MHz to +20 MHz, and accurate microwave pulse shaping for qubit operations, we demonstrate improved coherence times and reduced frequency crowding. These enhancements in qubit connectivity and control precision offer a scalable solution for quantum computing architectures, demonstrating the potential for extension to systems exceeding 50 qubits and advancing from error correction implementation.
Our findings not only represent a substantial step towards the realization of practical large-scale quantum computers but also provide a robust framework for further exploration of coherent and high-fidelity quantum operations. Future efforts will focus on scaling up the system size and refining error mitigation techniques.
Abstract
We report significant advancements in scalable quantum computing architecture using superconducting transmon qubits. Through implementation of a novel coupling scheme and improved control methods, we demonstrate enhanced multi-qubit operations in a 20-qubit processor while maintaining extended coherence times. The architecture features tunable coupling elements based on flux-biased SQUIDs, enabling dynamic control of inter-qubit interactions with coupling strengths ranging from -20 MHz to +20 MHz. Experimental results show average T1 relaxation times of 65 μs and T2 coherence times of 55 μs, with two-qubit gate fidelities reaching 99.2% and single-qubit gate fidelities of 99.8%. Our scalability analysis reveals linear control complexity scaling and minimal coherence degradation with increasing system size. These results represent a crucial step toward larger-scale quantum processors while maintaining high operational fidelities and coherence times necessary for practical quantum computation.
Keywords: quantum computing, superconducting qubits, scalable architecture, quantum coherence, gate fidelity
This paper reports advancements in scalable superconducting quantum computing architecture. We demonstrate a 20-qubit processor with improved coherence times and gate fidelities using a novel multi-qubit coupling scheme based on tunable flux-biased SQUIDs. We achieve average T1 and T2 times of 65 μs and 55 μs, respectively, and a two-qubit CNOT gate fidelity of 99.2% with gate times of 40 ns and crosstalk below 0.1%. The architecture exhibits linear scaling of control complexity while maintaining coherence and mitigating frequency crowding. These results pave the way for larger-scale, fault-tolerant quantum computers.
Abstract:
Title: Advances in Scalable Quantum Computing Architecture Using Superconducting Qubits
We report significant advances in scaling quantum computing architecture using superconducting transmon qubits, addressing key challenges in qubit connectivity and control. Our experimental results demonstrate a novel approach to scaling quantum systems while maintaining coherence times and gate fidelities. A 20-qubit processor featuring a two-dimensional lattice architecture with tunable couplers implemented using superconducting quantum interference devices (SQUIDs) exhibits improved multi-qubit coupling schemes and reduced crosstalk. We achieve average T1 relaxation times of 65 μs, T2 coherence times of 55 μs, and high-fidelity two-qubit gate operations with an average fidelity of 99.2% and crosstalk below 0.1%. Our architecture demonstrates linear scaling of control complexity, maintained coherence with increasing system size, and reduced frequency crowding. These results pave the way for larger-scale quantum processors and provide a foundation for future work on error correction protocols.
Keywords: superconducting qubits, quantum computing, scalability, coherence times, gate fidelity.
AnyModel: Explore and compare over 50 AI models side-by-side.
Budget Results
Title: Advances in Scalable Quantum Computing Architecture Using Superconducting Qubits
Abstract: Recent advancements in quantum computing architecture leveraging superconducting transmon qubits are presented, showcasing a significant step towards scalable and high-fidelity quantum systems. We demonstrate a novel architecture designed to facilitate the scaling of quantum processors while successfully preserving quantum coherence and gate fidelity. The experimental implementation features a 20-qubit processor arranged in a two-dimensional lattice, with enhanced multi-qubit coupling schemes and minimized crosstalk. Key findings include average T1 relaxation times of 65 μs and T2 coherence times of 55 μs, alongside impressive two-qubit gate fidelity of 99.2%. Our use of flux-biased superconducting quantum interference devices (SQUIDs) allows for dynamic, tunable coupling between qubits, significantly improving controllability and qubit connectivity. Scalability analysis indicates a linear increase in control complexity and sustained coherence with system expansion, paving the way for future quantum processors exceeding 50 qubits. These results underscore the viability of superconducting qubit systems for large-scale quantum computation and highlight pathways for implementing effective error correction protocols in subsequent research.
Advances in Scalable Quantum Computing Architecture Using Superconducting Qubits
This paper reports significant advancements in scalable superconducting quantum computing utilizing a 20-qubit transmon processor. A novel architecture featuring a two-dimensional lattice with tunable SQUID-based couplers achieves improved multi-qubit coupling and significantly reduced crosstalk (<0.1%). High coherence times (average T1 = 65 μs, T2 = 55 μs) and gate fidelities (single-qubit: 99.8%, two-qubit CNOT: 99.2%) were maintained despite the increased qubit count. This architecture demonstrates linear scaling of control complexity and mitigated frequency crowding, paving the way for the construction of larger-scale quantum processors. The results suggest a promising path towards fault-tolerant quantum computation.
Advances in Scalable Quantum Computing Architecture Using Superconducting Qubits
Abstract
Objective: Investigate novel architectural approaches for scaling quantum computing systems using superconducting transmon qubits while maintaining high coherence and gate performance.
Methods: We developed a 20-qubit processor with innovative multi-qubit coupling schemes utilizing flux-biased superconducting quantum interference devices (SQUIDs). The system employs a two-dimensional lattice configuration with dynamic coupling control and precise microwave pulse manipulation.
Results: The experimental quantum processor demonstrated exceptional performance metrics:
- Average coherence times (T2): 55 μs
- Two-qubit CNOT gate fidelity: 99.2%
- Crosstalk reduction: <0.1%
- Scalable control complexity with maintained quantum state preservation
Significance: This research provides a promising pathway for expanding quantum computing architectures, addressing critical challenges in qubit connectivity, coherence maintenance, and system scalability. The demonstrated techniques offer potential breakthroughs in developing larger, more stable quantum processors.
Keywords: Quantum computing, superconducting qubits, quantum architecture, scalable quantum systems
[Detailed paper continues as previously outlined]
Title: Advances in Scalable Quantum Computing Architecture Using Superconducting Qubits
Abstract:
We report a breakthrough in the development of scalable quantum computing architectures using superconducting transmon qubits. Our novel approach addresses key challenges in qubit connectivity and control, enabling the demonstration of a 20-qubit processor with improved multi-qubit coupling schemes and reduced crosstalk. Notably, we achieve average coherence times of 65 μs and 55 μs for T1
and T2
, respectively, in addition to a two-qubit controlled-NOT (CNOT) operation fidelity of 99.2%. Our results demonstrate linear scaling of control complexity, maintained coherence with increasing system size, and reduced frequency crowding, paving the way for large-scale quantum processors with high coherence and gate fidelities.
Keywords: quantum computing, superconducting circuits, transmon qubits, scalability, coherence times, gate fidelities.
Physical Review X, [Volume], [Issue], [Year], pp. [pages].
ArXiv: [ArXiv ID]
AnyModel: Explore and compare over 50 AI models side-by-side.
Performance Tier
ChatGPT 4 and Claude produced detailed, technical abstracts while Gemini and Llama offered more concise versions. Llama performed fastest (1282ms) but included unnecessary title and keywords. Gemini delivered the most efficient abstract (3430ms) with key metrics and findings without excess detail. ChatGPT (4895ms) and Claude (5028ms) were slowest but provided comprehensive coverage of methods, results and implications. For scientific abstract generation, Gemini appears optimal - balancing technical accuracy, conciseness and speed. All models demonstrated strong understanding of quantum computing terminology and proper abstract structure.
Budget Tier
The performance comparison shows distinct strengths across models. ChatGPT 4o Mini provided comprehensive technical details but was relatively slow (5.8s). Gemini 1.5 Flash delivered a concise, well-structured abstract with key metrics in just 3.9s. Claude 3.5 Haiku produced the most formally structured abstract with clear section headers, though took longest at 6.4s. Llama 3.1 8B was impressively fast (0.5s) while maintaining good technical accuracy and including academic formatting. Gemini appears optimal for this task, balancing speed with quality, while Llama excels if raw speed is priority.
Performance Verdict
After analyzing the performance across multiple abstract generation prompts, here is the comparative verdict for the leading AI models:
ChatGPT 4.0: Strengths:
- Most consistent in delivering well-structured, comprehensive abstracts
- Superior natural language flow and readability
- Excellent balance of technical detail and accessibility
- Strong performance on complex scientific and theoretical topics Weaknesses:
- Generally slower response times (4000-8000ms)
- Sometimes less specific with numerical data
Claude 3.5 Sonnet: Strengths:
- Best formatting and organization with clear headers
- Excellent inclusion of keywords and metadata
- Superior statistical detail and precision
- Strong scientific rigor Weaknesses:
- Slowest average response times (5000-10000ms)
- Sometimes overly detailed for abstract format
Gemini 1.5 Pro: Strengths:
- Most comprehensive technical and statistical data
- Excellent handling of scientific terminology
- Good balance of detail and efficiency
- Strong performance metrics presentation Weaknesses:
- Often lacks clear paragraph breaks
- Can be overly detailed and dense
- Sometimes needs better organization
Llama 3.1 70B: Strengths:
- Consistently fastest response times (700-2000ms)
- Good inclusion of formatting elements
- Efficient delivery of key information
- Strong opening context Weaknesses:
- Less detailed statistical information
- Sometimes includes unnecessary elements
- Can be overly lengthy
Overall Winner: ChatGPT 4.0
While each model showed distinct strengths, ChatGPT 4.0 emerges as the most reliable and balanced performer for abstract generation. It consistently delivers well-structured, comprehensive abstracts with appropriate technical detail and natural language flow across diverse topics. Though not the fastest, its superior quality and consistency make it the top choice for this use case. Gemini 1.5 Pro deserves an honorable mention for technical accuracy and efficiency, while Llama 3.1 70B stands out for speed-critical applications.
Budget Verdict
Based on the comparative analyses of ChatGPT 4o Mini, Claude 3.5 Haiku, Gemini 1.5 Flash, and Llama 3.1 8B in generating abstracts across various academic fields, here's the comprehensive performance verdict:
Processing Speed:
- Llama 3.1 8B consistently demonstrated superior speed, averaging 400-700ms per response
- Other models typically required 5-15 seconds
- Speed ranking: Llama 3.1 8B > ChatGPT 4o Mini > Claude 3.5 Haiku > Gemini 1.5 Flash
Content Quality:
- Claude 3.5 Haiku excelled in academic formatting and organization
- Gemini 1.5 Flash provided optimal balance of conciseness and technical depth
- ChatGPT 4o Mini offered comprehensive but sometimes verbose content
- Llama 3.1 8B delivered good technical accuracy but less sophisticated organization
Strengths by Model: ChatGPT 4o Mini:
- Comprehensive technical detail
- Strong statistical presentation
- Well-structured responses
Claude 3.5 Haiku:
- Superior academic formatting
- Clear section headers
- Excellent organization
- Best keyword implementation
Gemini 1.5 Flash:
- Optimal balance of brevity and depth
- Precise technical details
- Comprehensive coverage of methodology
- Consistent quality across subjects
Llama 3.1 8B:
- Exceptional processing speed
- Good technical accuracy
- Efficient summarization
- Best speed-to-quality ratio
Weaknesses by Model: ChatGPT 4o Mini:
- Tendency toward verbosity
- Slower processing times
- Sometimes overly detailed
Claude 3.5 Haiku:
- Slowest processing times
- Sometimes over-structured
- Excessive formatting for simple abstracts
Gemini 1.5 Flash:
- Longest processing times
- Less formal structure
- Inconsistent formatting
Llama 3.1 8B:
- Less sophisticated organization
- Limited statistical detail
- Less formal academic structure
Overall Winner: Gemini 1.5 Flash
While Llama 3.1 8B impressed with speed and Haiku excelled in formatting, Gemini 1.5 Flash consistently delivered the best balance of comprehensive content, technical accuracy, and appropriate length across all subject matters. Its ability to maintain high-quality output while providing precise technical details and methodology makes it the most reliable choice for abstract generation, despite longer processing times.
Conclusion
Conclusion
After extensive testing and analysis of both performance and budget tier AI models for abstract generation, several key insights emerge. While the performance tier models demonstrated superior capabilities in handling complex technical content and maintaining academic rigor, the budget tier alternatives proved remarkably capable for many standard abstract writing tasks.
The performance tier analysis crowned ChatGPT 4.0 as the overall winner, excelling in consistency, structure, and natural language flow. However, each model showed distinct advantages - Claude 3.5 Sonnet for formatting, Gemini 1.5 Pro for technical depth, and Llama 3.1 70B for processing speed.
In the budget tier, Gemini 1.5 Flash emerged victorious by delivering the optimal balance of comprehensive content and technical accuracy, despite slower processing times. Llama 3.1 8B impressed with exceptional speed, while Claude 3.5 Haiku stood out for superior formatting and organization.
The choice between tiers ultimately depends on specific needs: Performance tier models are ideal for complex scientific papers and critical academic work requiring highest accuracy, while budget tier options prove sufficient for standard abstract generation tasks where cost-efficiency is paramount. This evaluation demonstrates that recent advances in AI have made even budget-friendly models viable alternatives for many academic writing applications.